Improved Faster R-CNN Algorithm for Robust Detection of Tank Targets in Complex Environments
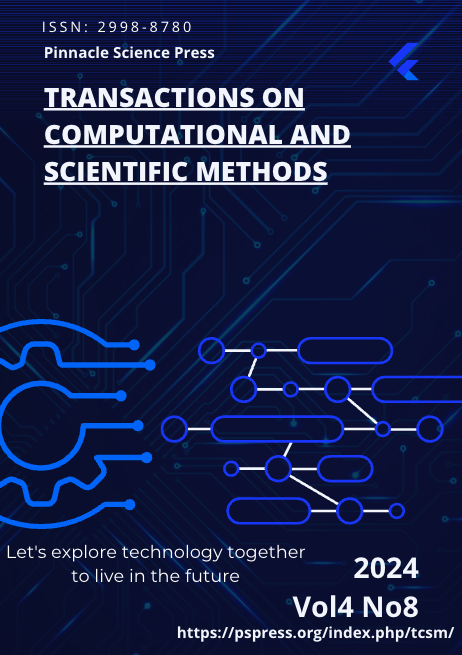
Abstract
Target detection plays a vital role in various fields, including military applications, where the detection of tank targets is crucial for accurate battlefield assessments. However, the complexity of modern combat environments, including target occlusion and camouflage, presents significant challenges. Traditional target detection algorithms, once dominant, have been surpassed by deep learning methods, particularly convolutional neural networks (CNNs). This paper explores the application of the Faster R-CNN algorithm for tank target detection, focusing on enhancing detection accuracy and robustness in complex settings. To address the issue of target occlusion and limited data, the Faster R-CNN model is improved by integrating a ResNet50 backbone with a Feature Pyramid Network (FPN) for more refined feature extraction.