K-Means and N-TSK Algorithm for EEG-Based Alzheimer’s Disease Feature Identification
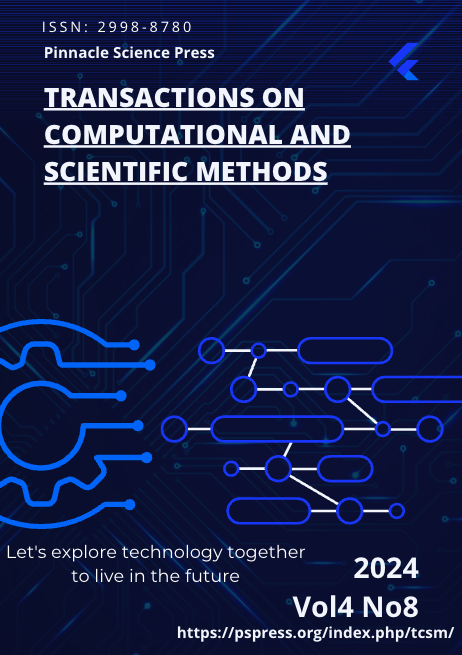
Abstract
Alzheimer's Disease (AD) is a prevalent neurodegenerative condition characterized by progressive cognitive and memory decline, significantly impairing daily living activities. Despite advancements in medicine, effective treatments remain elusive. This study explores the application of the N-TSK algorithm to identify features in EEG brain function networks of Alzheimer’s patients, combining complex network analysis with a Takagi-Sugeno-Kang (TSK) fuzzy system model to improve identification accuracy and interpretability. While this approach enhances model performance, subjectivity in determining the index weight vector poses challenges, particularly when the feature set is large. Additionally, K-means clustering was employed due to its simplicity and efficiency in clustering EEG data. However, the algorithm’s limitation lies in the need for prior knowledge to preset the appropriate K value, as random selection can yield unsatisfactory clustering results. Further research is needed to refine these methods for more accurate and scientific clustering in Alzheimer's disease diagnosis.