Transformers in Opinion Mining: Addressing Semantic Complexity and Model Challenges in NLP
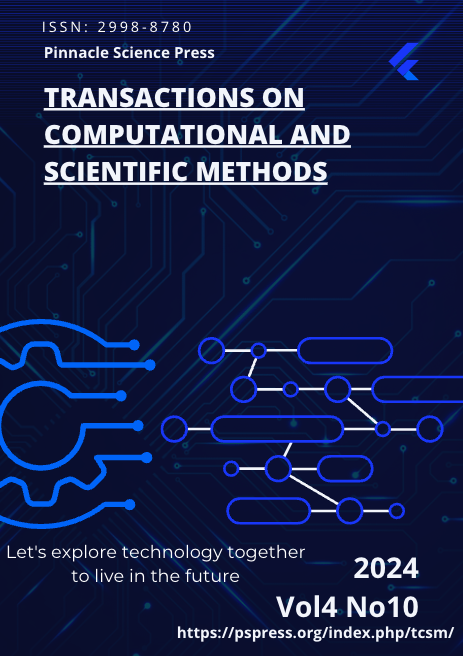
Abstract
With the rapid development of natural language processing (NLP) technology, models based on the Transformer architecture have become one of the mainstream methods in the field of NLP due to their excellent performance. By introducing the self-attention mechanism, Transformer can more effectively capture long-distance dependencies in text, and has made breakthrough progress in a series of tasks such as machine translation, sentiment analysis, and text summarization. Opinion mining, as an important branch of NLP, aims to automatically identify and extract subjective information from a large amount of unstructured data, such as opinions and attitudes in product reviews or social media posts. The opinion mining system combined with Transformer can not only help companies better understand consumer needs, but also provide support for public opinion monitoring for government agencies, and has broad application prospects. In practical applications, traditional opinion mining technology faces many challenges, such as limited semantic understanding ability and difficulties in processing complex sentence structures. However, with the help of Transformer's powerful representation learning ability, these problems have been alleviated to a certain extent, especially when faced with information containing irony or metaphorical expressions, the Transformer-based method can more accurately grasp the author's true intentions.