A Review of Smoke Detection Techniques Using Deep Learning and Image Processing: Challenges and Future Directions
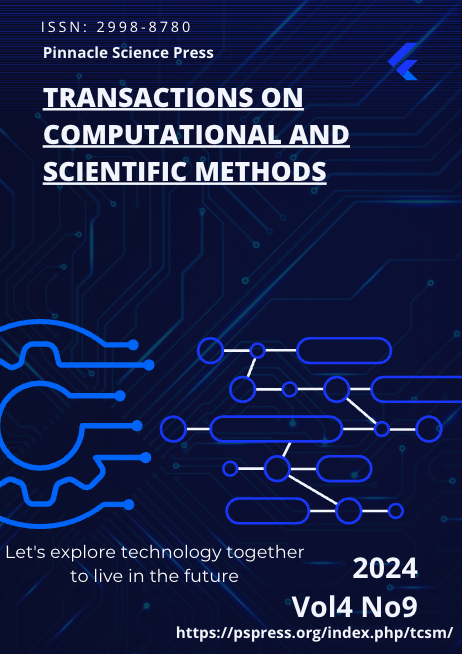
Abstract
Traditional smoke detection methods, relying on sensors for temperature, smoke, and light changes, face limitations in large or outdoor environments due to their dependency on proximity to combustion by-products. While early image processing techniques improved detection accuracy for complex spaces, they were constrained by manually set thresholds and shallow feature extraction, resulting in high false detection rates and poor generalization. The advent of deep learning has transformed smoke detection, allowing for automatic feature extraction and improved accuracy. This paper reviews the progress of deep learning techniques applied to smoke detection, highlighting convolutional neural networks (CNNs) and their effectiveness in capturing static smoke features. Despite these advances, challenges remain in detecting dynamic smoke features, especially in complex environments. Future research directions include the simultaneous extraction of smoke color and texture features, the use of multi-scale optical flow and velocity distortion to track smoke dynamics, and the integration of CNNs with support vector machine classifiers to enhance detection accuracy in diverse conditions.