Enhancing Entity Relationship Extraction Using Duie_Bert and Improved Cascading Pointer Networks
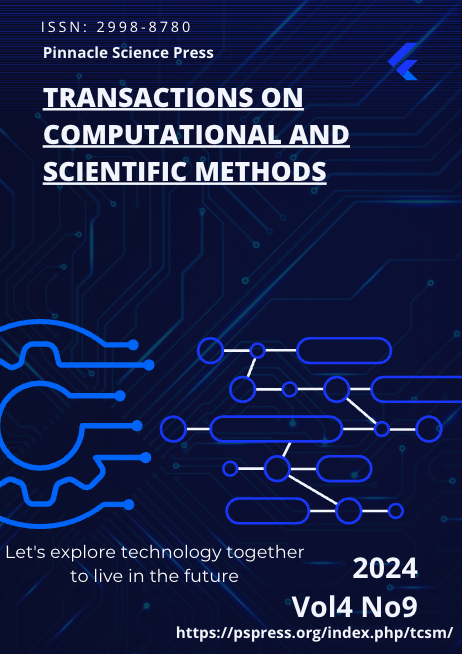
Abstract
Relationship extraction plays a pivotal role in building knowledge graphs, especially in the era of big data, where efficiently extracting entity-relationship triples from vast unstructured text is a growing challenge. Traditional feature engineering approaches face limitations such as ambiguity and redundancy. Recent advancements in deep learning, particularly the use of neural networks, have significantly improved entity relationship extraction. This paper proposes a novel approach incorporating the Duie_Bert pre-training model and an improved cascading pointer network, designed to address issues related to overlapping relational triples and data miscommunication. A multi-headed attention mechanism guided by specific relation-entity vectors enhances the feature representation, improving the accuracy of relational extraction. Experimental results on the Duie Chinese relational dataset demonstrate that the proposed model surpasses existing methods, achieving a 9.0% increase in recall and a 5.2% improvement in F1 score. Future work will focus on domain-specific knowledge graph construction using the proposed entity-relationship extraction model.