Panoramic Image Segmentation Using Attention Mechanism with ResNet-50 Backbone and Multi-Task Learning
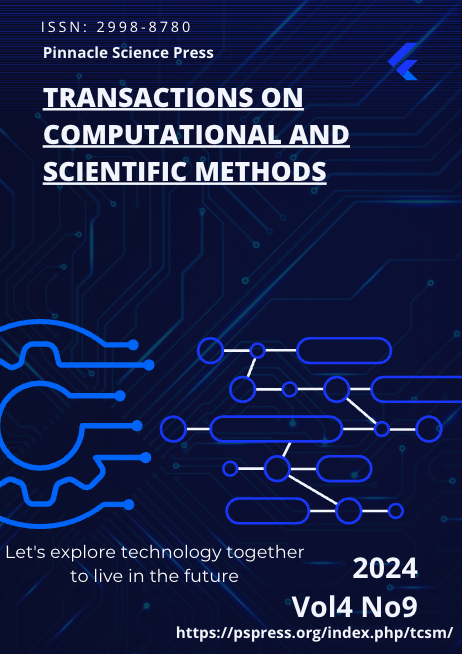
Abstract
Traditional image segmentation techniques, such as semantic and instance segmentation, often fall short in providing comprehensive scene understanding. Semantic segmentation fails to differentiate individual objects within the same category, while instance segmentation cannot identify distinct background regions. To address these limitations, panoramic segmentation was introduced, combining the strengths of both methods to assign semantic categories to each pixel while distinguishing objects of the same category. This paper proposes an improved panoramic segmentation approach based on attention mechanisms. Using ResNet-50 as the backbone, the method extracts features that are processed separately by semantic and instance segmentation branches. The semantic segmentation branch employs a fully convolutional network (FCN), while the instance segmentation branch uses Mask R-CNN, with information flow shared between branches. A cross-layer attention fusion module aggregates multi-scale features into a prototype mask module to enhance segmentation accuracy. The final results from both branches are fused heuristically to produce refined panoramic segmentation output, effectively addressing occlusion and improving scene comprehension.