An Enhanced MRI Image Segmentation Approach for Brain Tumors Using SEVNet3D
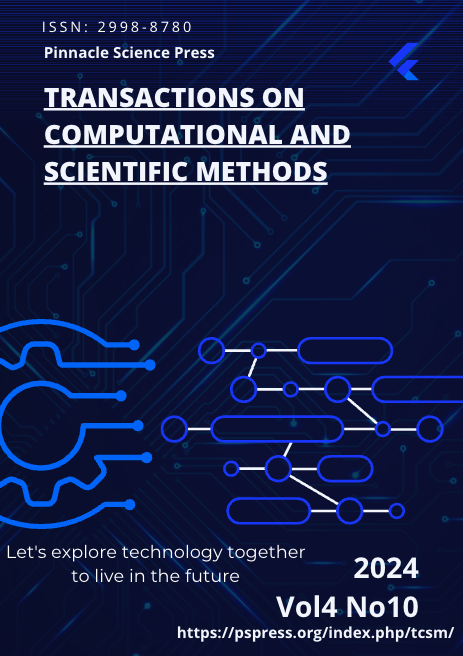
Abstract
Brain tumors are a significant medical concern due to their high morbidity and mortality rates, comprising 2.4% of all human tumors. Accurate segmentation of brain tumors in magnetic resonance imaging (MRI) is crucial for effective diagnosis and treatment planning. Traditional image segmentation methods are often limited by their reliance on shallow image features and sensitivity to parameter settings. Deep learning-based segmentation techniques, while powerful, face challenges such as high computational complexity, limited accuracy, and insufficient contextual information integration. In this study, we propose an improved segmentation model, SEVNet3D, which incorporates Squeeze-and-Excitation (SE) modules to enhance the performance of the VNet architecture. The SEVNet3D model achieves better generalization and segmentation accuracy with minimal computational overhead. Despite its advantages, challenges such as the intensive workload of sample annotation and long segmentation times remain. Future work will focus on optimizing the algorithm to enable rapid segmentation and facilitate efficient diagnosis by medical professionals.