Leveraging Machine Learning for Stock Market Prediction: A Comprehensive Analysis of Market Trends and Practical Applications
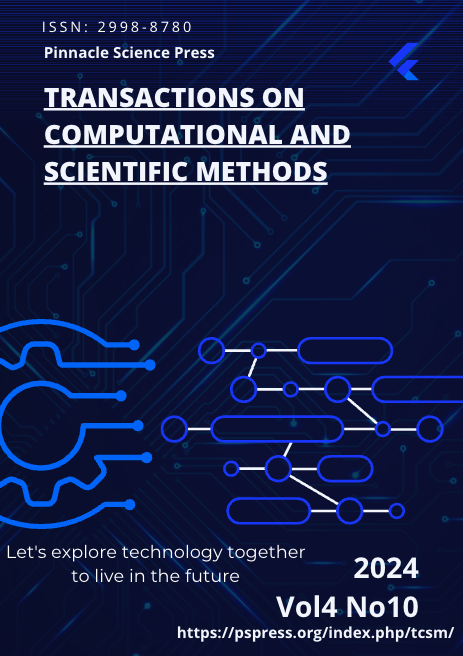
Abstract
The rapid advancements in big data and artificial intelligence have significantly reshaped financial markets, particularly in stock price forecasting. This study delves into the increasing influence of big data on the Chinese stock market, emphasizing the role of machine learning algorithms in enhancing prediction accuracy and decision-making efficiency. Beginning with an exploration of China's stock market evolution and the strategic importance of big data, the paper highlights the challenges traditional financial models face in navigating the nonlinear complexities of stock price trends. By analyzing existing algorithms, such as artificial neural networks and support vector machines (SVM), the research underscores their advantages and limitations, particularly in addressing noisy and multidimensional data. The study further proposes a novel voting method that combines feature selection with classifier optimization to enhance prediction performance. While machine learning shows promise in improving stock price trend predictions and revenue certainty, the paper also identifies limitations stemming from data dependency and the diverse economic factors influencing market behaviors. Recommendations for future research include expanding data sets, refining industry-specific analyses, and adapting machine learning models to dynamic trading scenarios. This work aims to bridge theoretical insights and practical applications, contributing to the broader discourse on financial technology integration in stock market forecasting.