Personalized Multimodal Recommendations Framework Using Contrastive Learning
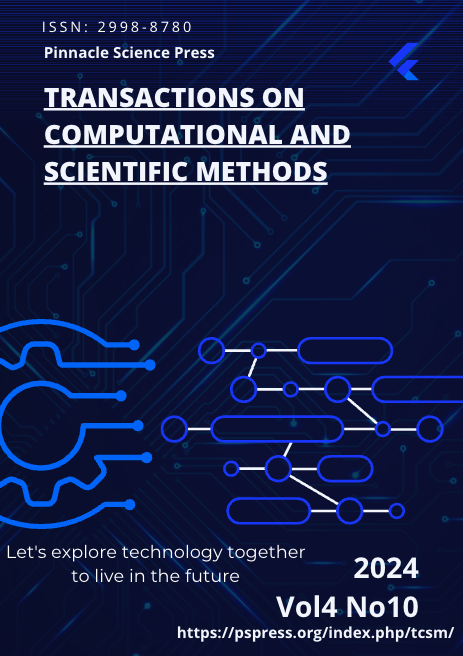
Abstract
This study proposes a multimodal cross-domain recommendation framework based on contrastive learning to address the shortcomings of traditional recommendation systems in dealing with multimodal data and cross-domain user preferences. By encoding multimodal features such as text and images of users and items, and using contrastive learning strategies to construct positive and negative sample pairs, the framework can effectively capture the associations between different modalities in high-dimensional feature space. In addition, through the cross-domain alignment method, the migration and integration of user preferences are achieved, so that the model can adapt to the complex needs of users in multiple fields. Experimental results show that the framework outperforms traditional models in evaluation indicators such as NDCG and AUC, and shows high accuracy and robustness in dealing with cross-domain recommendation tasks. This study verifies the application potential of contrastive learning in recommendation systems and provides a new technical approach for future recommendation systems. At the same time, this paper compares and analyzes a variety of classic recommendation models, further highlighting the role of cross-domain information and multimodal fusion in improving recommendation effects. Future research directions include improving contrastive learning methods, exploring the use of more unsupervised data, and improving the real-time response capability of the model, so as to provide users with better personalized recommendation services.
Keywords
Contrastive learning, multimodal recommendation, cross-domain recommendation, personalized recommendation