Advances and Challenges in Deep Learning-Based Medical Image Segmentation
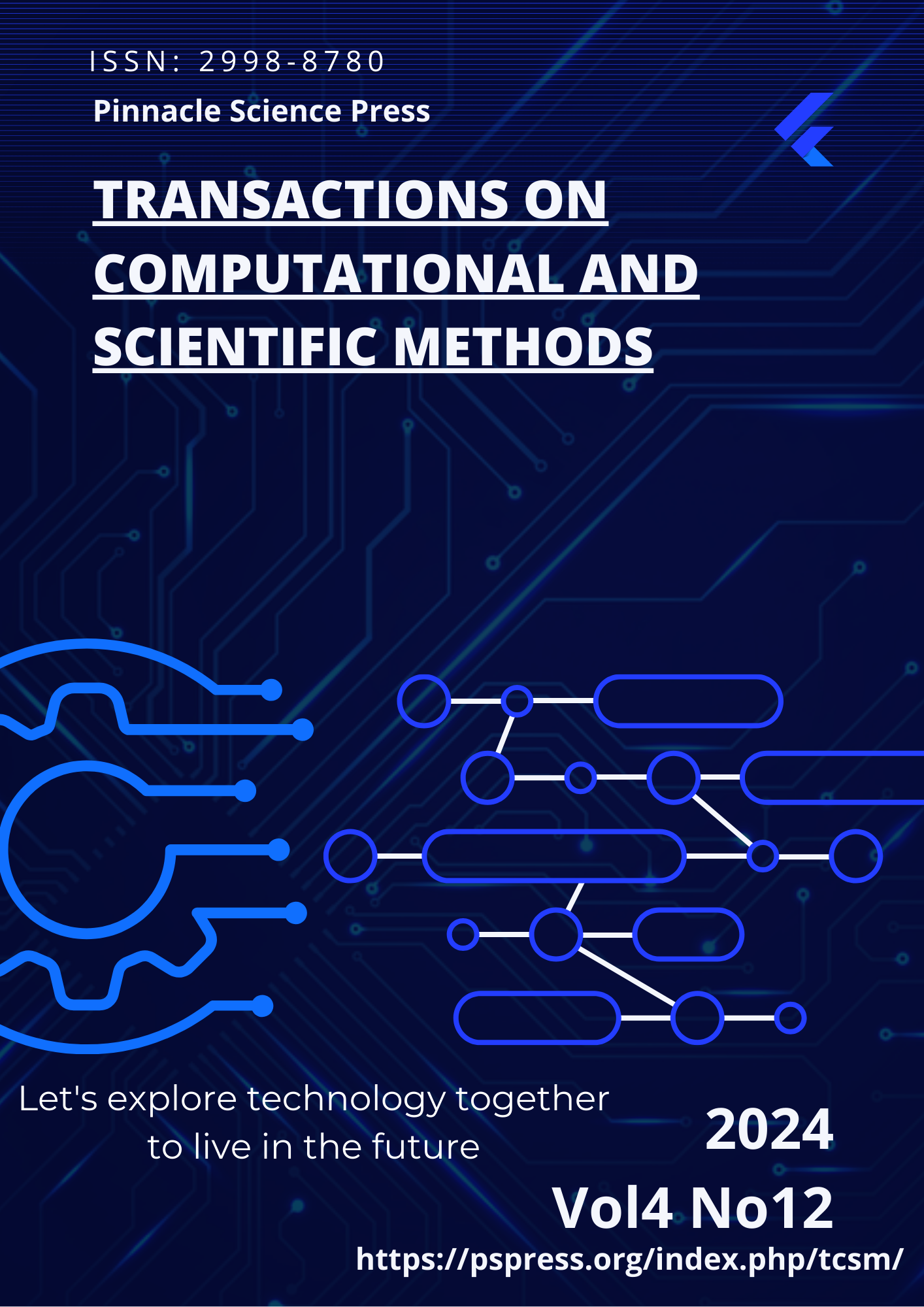
Abstract
The rapid growth of medical image data presents significant challenges for clinicians while offering opportunities to revolutionize diagnostic and treatment practices. Leveraging deep learning technologies, medical image segmentation has emerged as a critical tool for isolating regions of interest (ROI), enabling precise diagnosis and treatment planning. Compared to traditional segmentation methods, deep learning-based approaches eliminate the need for human intervention and deliver superior accuracy and efficiency. However, challenges persist, including limitations in processing high-resolution images, scarcity of annotated medical datasets, and risks of model overfitting due to small-scale training data. Promising research directions include semi-supervised and unsupervised segmentation, as well as the application of generative adversarial networks (GANs) to augment training datasets. These innovations, coupled with advances in hardware and algorithm development, are poised to address existing limitations and significantly enhance medical image segmentation's role in disease diagnosis and management.