Artificial Intelligence-Driven Risk Assessment and Control in Financial Derivatives: Exploring Deep Learning and Ensemble Models
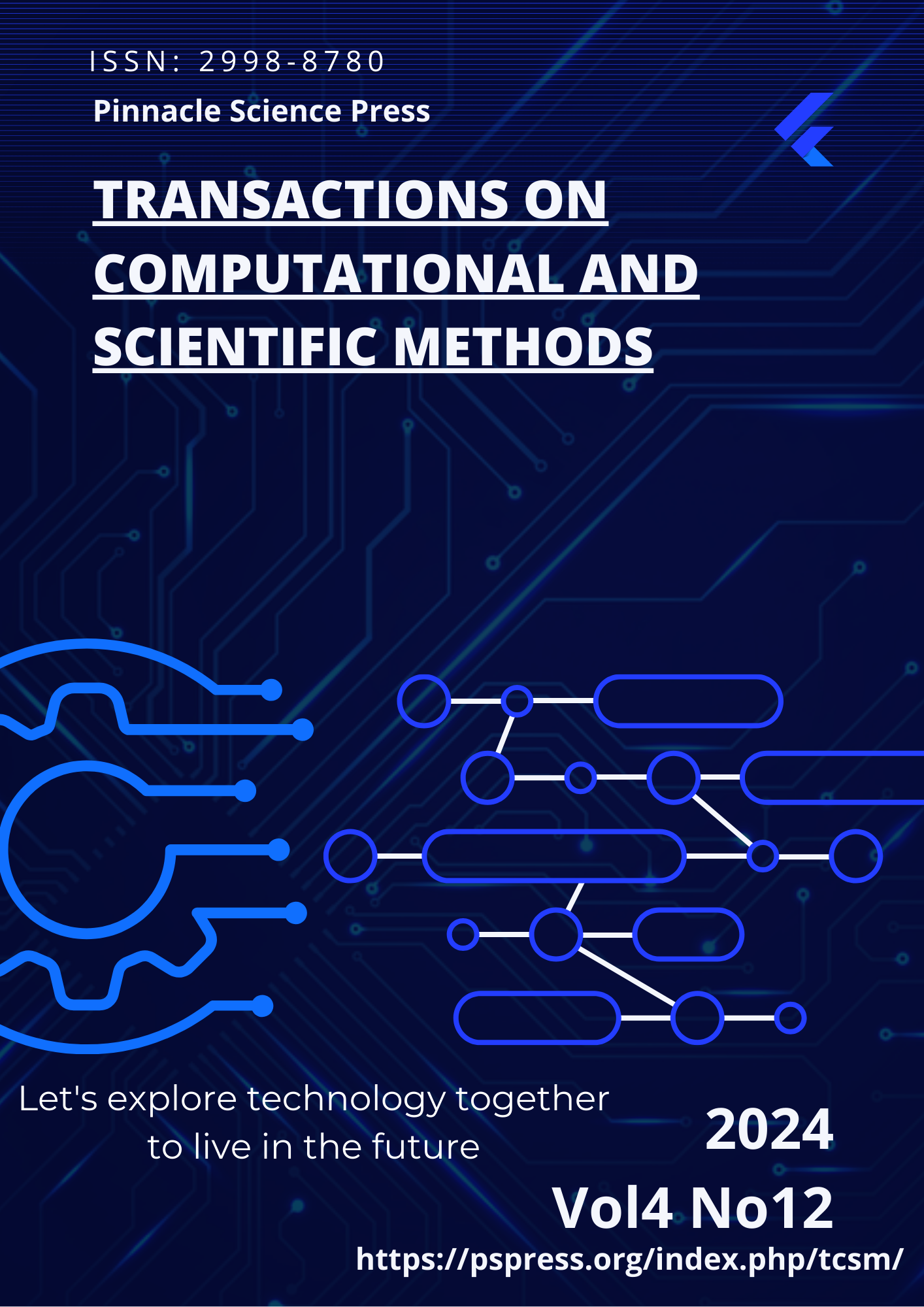
Abstract
The rapid development of artificial intelligence technology has brought new solutions to the risk control of financial derivatives. Financial derivatives face huge challenges in market risk and credit risk management due to their complex structure and high leverage characteristics. Traditional risk management methods are difficult to adapt to high-frequency and nonlinear data environments due to their reliance on linear assumptions and the limitations of market dynamics. This paper focuses on the application of artificial intelligence in the assessment of market risk and credit risk of financial derivatives, and explores the potential of deep learning models and ensemble learning algorithms in risk prediction. By introducing models such as long short-term memory network (LSTM), temporal convolutional network (TCN), logistic regression, support vector machine (SVM) and gradient boosting tree (XGBoost), the applicability and performance of different models in financial data modeling are analyzed. The study shows that deep learning models can handle complex time series features, and ensemble learning algorithms have high prediction accuracy and stability in credit risk identification. In the future, with the deep integration of artificial intelligence algorithms and financial market data, intelligent risk management systems will have stronger real-time response capabilities and decision support functions, providing important support for the stable operation of financial markets and investor protection.