Dynamic Risk Control and Asset Allocation Using Q-Learning in Financial Markets
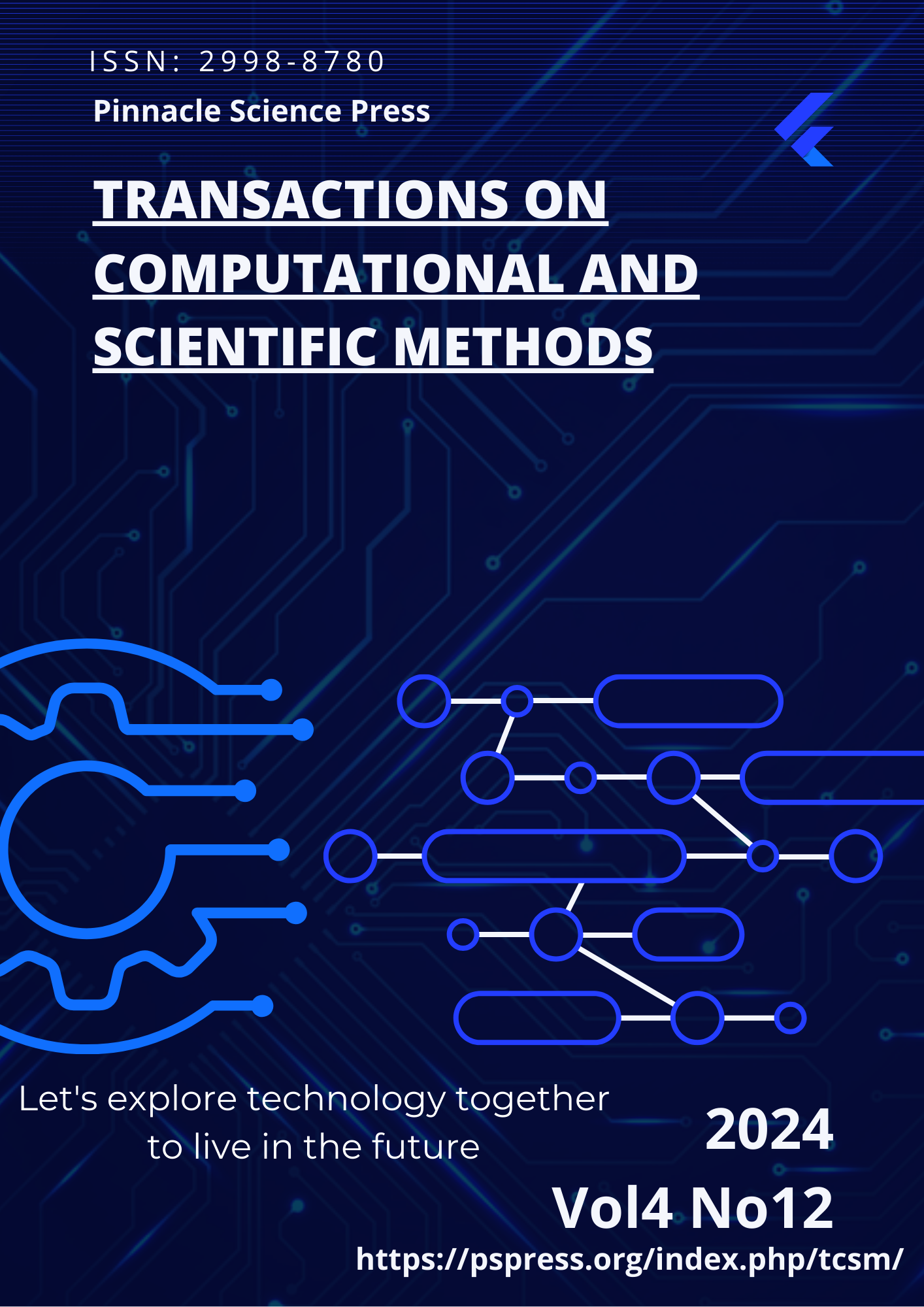
Abstract
This study proposes an asset management risk control algorithm based on Q-learning, which aims to optimize asset allocation decisions through reinforcement learning, maximize investment returns, and effectively control risks. We used a real financial market dataset from Yahoo Finance to train and verify the algorithm. The dataset contains historical closing prices, trading volumes, volatility, and other information of multiple assets, with a time span of 5 years and a data frequency of daily. Experimental results show that Q-learning outperforms traditional models such as mean-variance optimization (MVO), genetic algorithm (GA), deep Q network (DQN), and support vector machine (SVM) in multiple evaluation indicators. Specifically, Q-learning achieved the best results in indicators such as cumulative return, Sharpe ratio, and maximum drawdown, demonstrating its adaptability and efficiency in a dynamic market environment. By simulating investment decisions under different market conditions, the Q-learning algorithm can adaptively adjust investment strategies, effectively respond to market fluctuations, and achieve optimal risk control. Despite this, reinforcement learning algorithms still have certain challenges in computational complexity and training time. In the future, the computational efficiency of the model can be improved by introducing more efficient algorithms and optimization strategies.