Efficient Underwater Biological Target Detection Using the YOLOv7-Tiny Algorithm
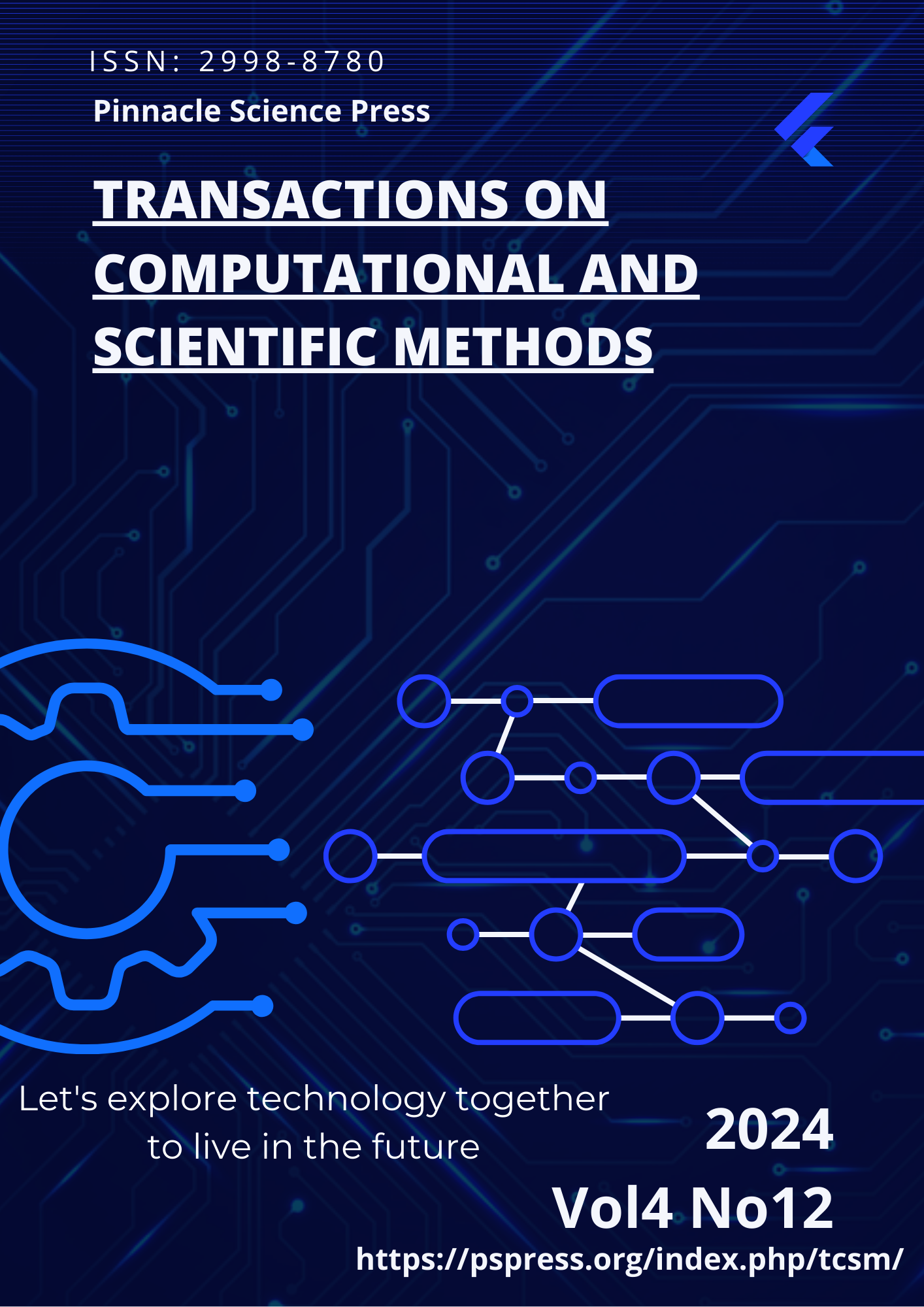
Abstract
The detection and recognition of underwater organisms are critical for marine resource utilization and economic development. This study introduces a YOLOv7-tiny-based target detection algorithm optimized for underwater biological target detection. Trained on the Aquarium marine organism dataset, the proposed algorithm is evaluated against YOLOv5s, demonstrating improved accuracy, recall, and average precision while significantly reducing model size and parameter count. These improvements enhance its suitability for deployment on embedded devices in complex underwater environments. Future work will focus on integrating attention mechanisms, small target detection layers, and novel loss functions to broaden the algorithm's applicability across diverse domains.