Enhancing Recommendation Systems through a Multi-Modal Knowledge Graph Neural Network Model
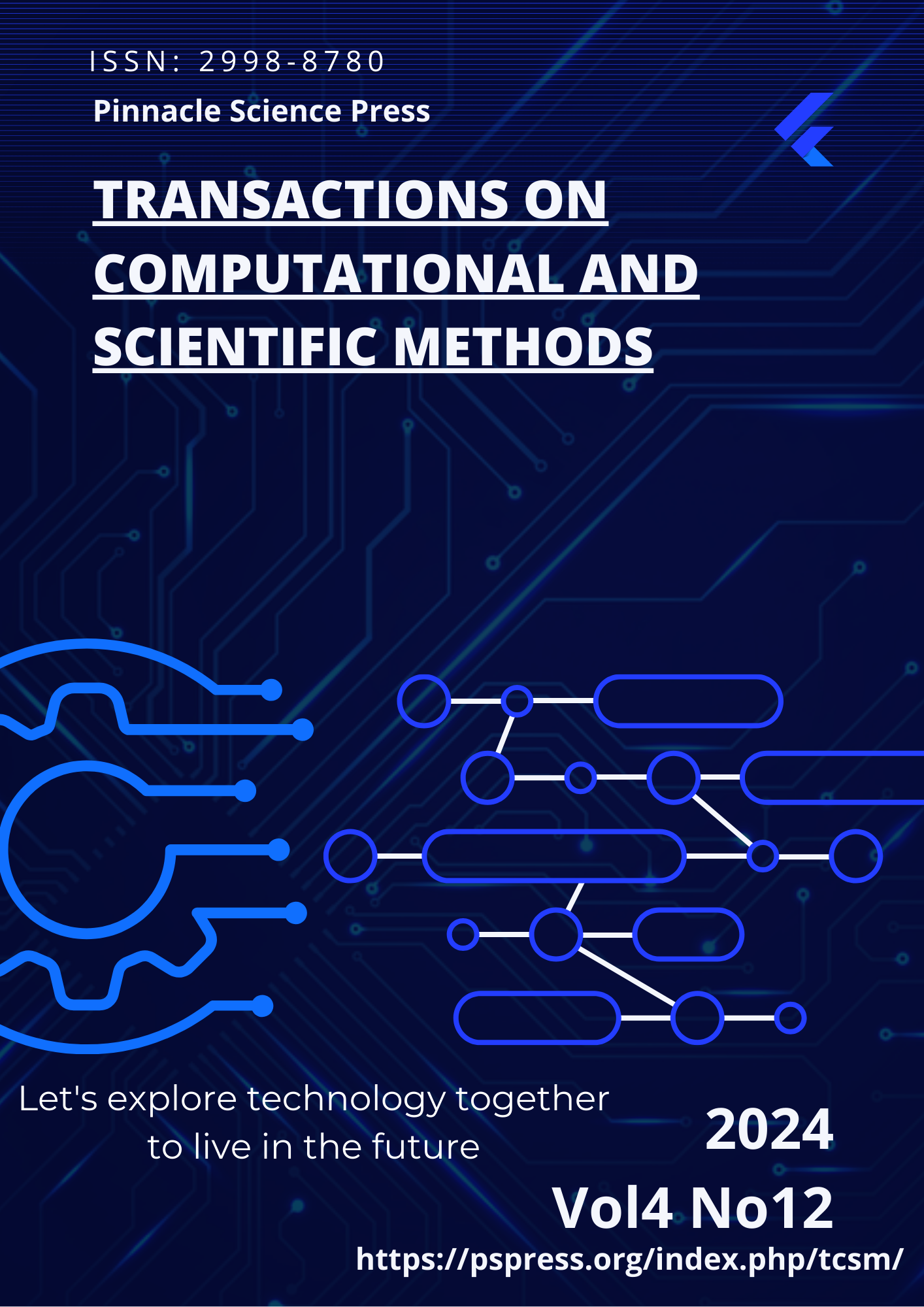
Abstract
With the rapid development of artificial intelligence, recommendation systems play a crucial role across various domains such as entertainment, e-commerce, and social networking. Collaborative filtering has traditionally been the cornerstone of recommendation algorithms but faces limitations due to data sparsity and the cold start problem. In recent years, the introduction of knowledge graphs has enhanced recommendation systems by providing structured auxiliary information; however, these models typically rely solely on text-based data, overlooking other valuable forms of information such as images, audio, and video. This study addresses this gap by proposing a recommendation model based on a multi-modal knowledge graph neural network (MGNN), integrating text, visual, and audio data to create a more comprehensive and dimensional knowledge representation. Focusing on the domain of movie recommendations, we construct a multi-modal knowledge graph and employ the MGNN model to fuse features from diverse data types. This enables the extraction and aggregation of multi-modal attributes, thereby enhancing recommendation accuracy and system performance. Experimental results demonstrate that the multi-modal knowledge graph approach substantially outperforms traditional recommendation systems. This research contributes to the fields of multi-modal data integration and knowledge graph-enhanced recommendation systems, and lays groundwork for further advancements in multi-modal recommendation methodologies.