Facial Emotion Recognition Using an Enhanced Xception Network for Behavioral Analysis
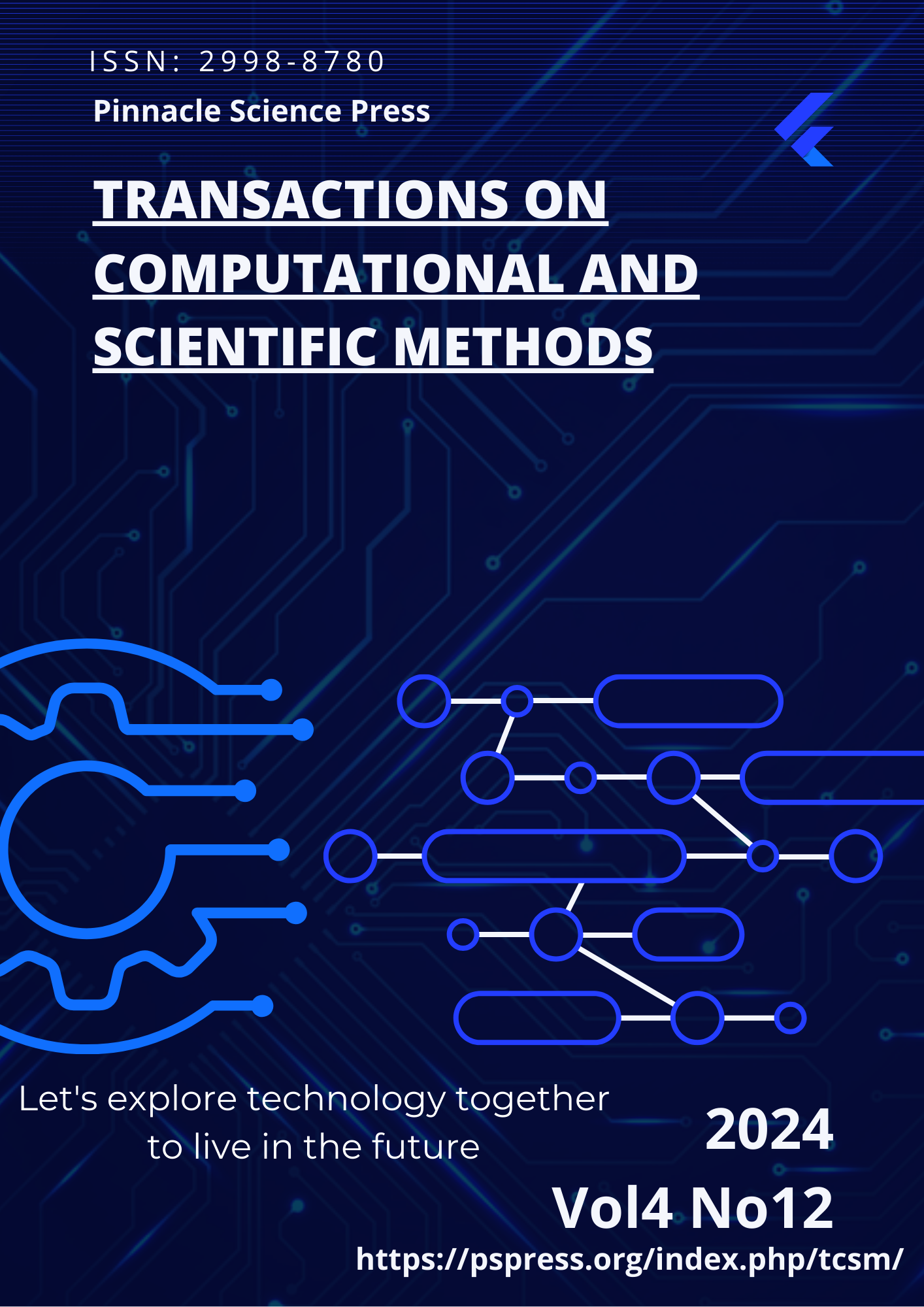
Abstract
The rise in mental health issues such as depression and anxiety has necessitated efficient diagnostic tools. This study integrates facial recognition with psychological analysis, utilizing deep learning techniques to achieve accurate emotion recognition. An improved Xception network was developed, featuring densely connected depthwise separable convolution modules to optimize parameter usage and reduce computational demands, making it suitable for mobile applications. The model employs the FSRNet hypernetwork for facial feature extraction and multi-scale feature learning, achieving over 95% accuracy in facial emotion recognition. This approach provides a foundation for mental health prediction and diagnosis, significantly reducing the time and cost of psychological evaluations. Future research will focus on enhancing detection speed and extending functionality across platforms for broader applications in mental health analysis.