Fully Convolutional Neural Networks for High-Precision Medical Image Analysis
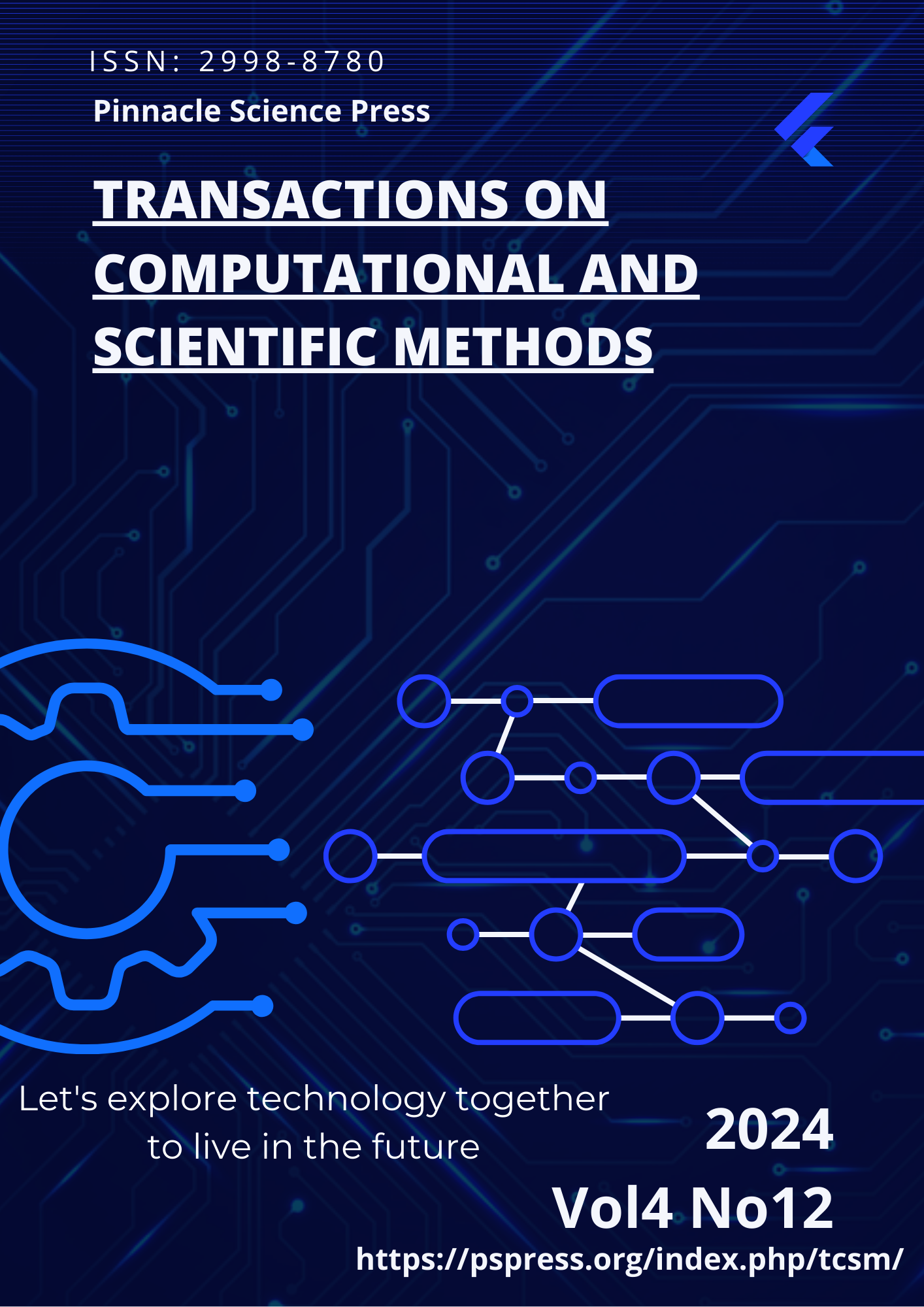
Abstract
This study proposed a medical image semantic segmentation algorithm based on a fully convolutional neural network (FCNN) for accurate segmentation of anatomical structures and lesion areas in medical images. In the experiment, we used mIOU and Dice coefficients as the main evaluation indicators to verify the superior performance of FCNN in fine-grained segmentation tasks. The experimental results show that FCNN performs well in both mIOU and Dice values, surpassing traditional semantic segmentation models such as U-Net, SegNet, and DeepLabV3. This shows that the convolutional structure and end-to-end feature extraction mechanism of FCNN can effectively capture the complex boundaries and subtle features in medical images and achieve high-precision pixel-level segmentation. The algorithm has good generalization and strong cross-modal adaptability, and is suitable for a variety of medical image types such as CT and MRI. In the future, with the development of deep learning technology, the application potential of FCNN in the field of medical image analysis will be further expanded, providing technical support for precision medicine and intelligent diagnosis and treatment, and providing auxiliary decision-making bases for medical workers.