Performance Evaluation and Insights into Supervised Learning Models for Single-Cell RNA-Seq Data Classification
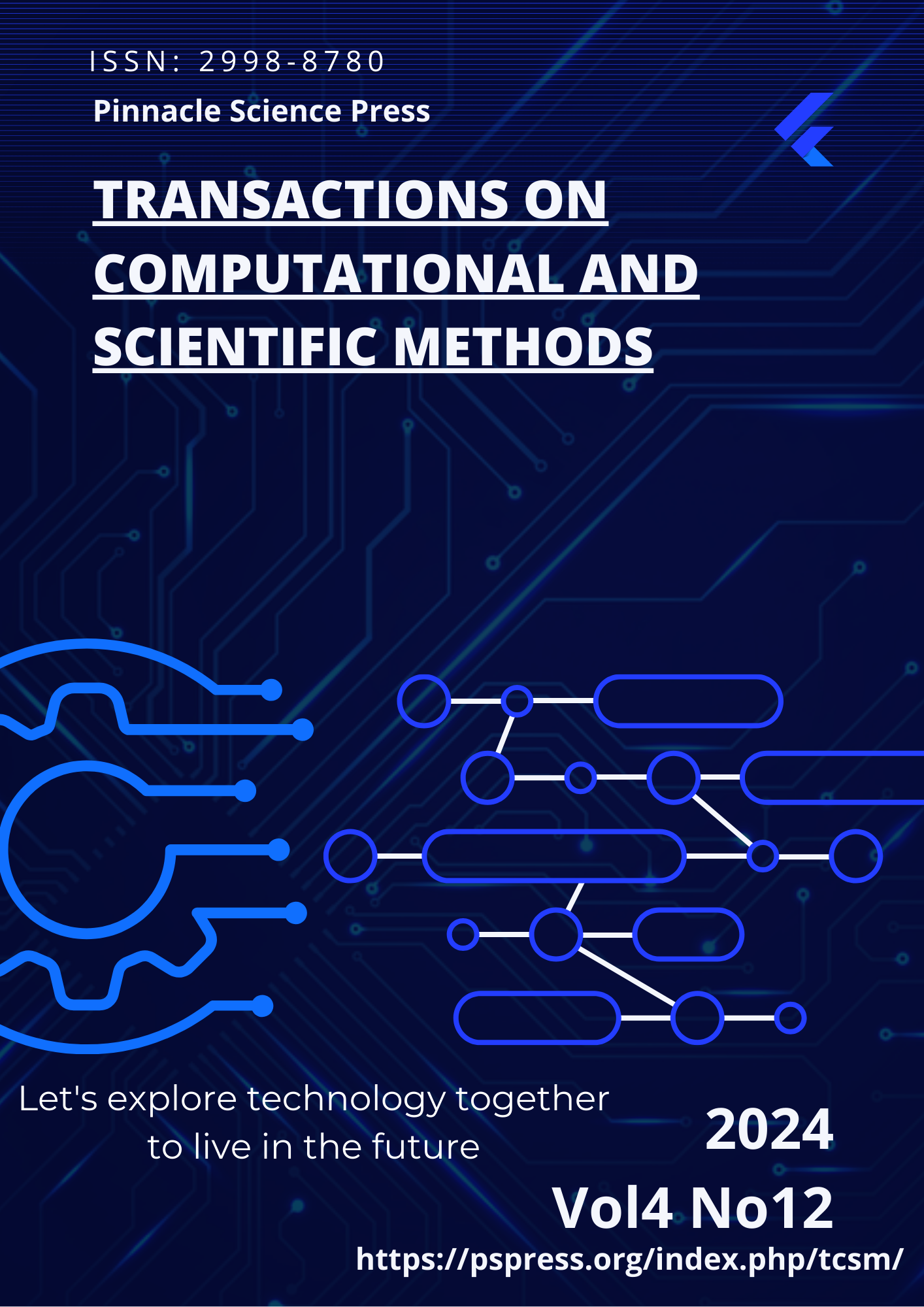
Abstract
Single-cell RNA-sequencing (scRNA-seq) technology enables precise measurement of gene expression at the single-cell level, offering insights into cell subpopulations that bulk RNA sequencing cannot provide. However, effective classification of scRNA-seq data remains a challenge due to its high-dimensional, batch-variable, and complex nature. In this study, we empirically evaluate the performance of four supervised learning models—decision trees (DT), random forests (RF), boosting, and logistic regression (LR)—on scRNA-seq data. While decision tree-based methods have traditionally shown strong performance in gene expression analysis, our results reveal that logistic regression outperformed the other models in terms of accuracy. This suggests that LR provides a robust and interpretable solution for cell-type classification in scRNA-seq data. Despite its effectiveness, the model's performance is limited by the available training data and diversity of cell types. Future research should address these limitations through expanded datasets, further empirical evaluations, and integration of advanced ensemble techniques for improved classification performance.