A CatBoost-Driven Model for Predicting and Analyzing Market Price Trends
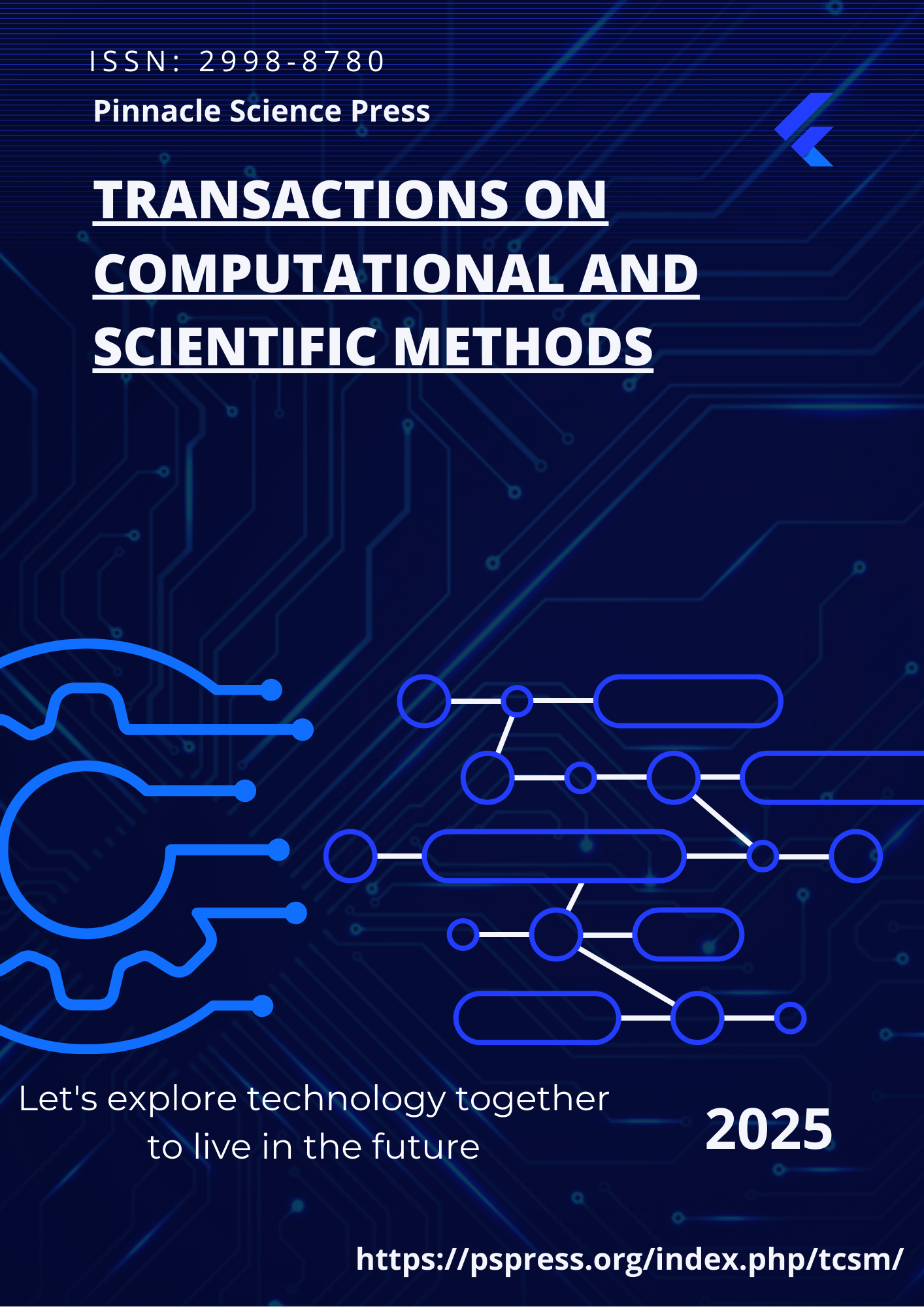
Abstract
Accurate prediction of building material prices is crucial for mitigating the impacts of cost fluctuations on project budgets, schedules, and quality. With rising competition and price volatility in the construction industry, traditional prediction models often face challenges such as slow training speeds and high sensitivity to parameter tuning. This study proposes a building material price prediction model utilizing the CatBoost algorithm, a gradient boosting framework optimized for categorical features and robust prediction performance. Using sample data of Φ16-25mm HRB400 type bar steel material prices and relevant influencing factors, the model is evaluated against common machine learning algorithms, including XGBoost and LightGBM. Experimental results demonstrate that the CatBoost-based model outperforms these alternatives in terms of mean squared error (MSE), root mean squared error (RMSE), mean absolute error (MAE), mean absolute percentage error (MAPE), and R². The findings highlight the superior prediction accuracy and generalization ability of the proposed model, offering valuable insights for construction cost control and resource planning. Future research will explore broader applications and refine the model to adapt to diverse market dynamics.