Deep Learning-Based Gesture Key Point Detection for Human-Computer Interaction Applications
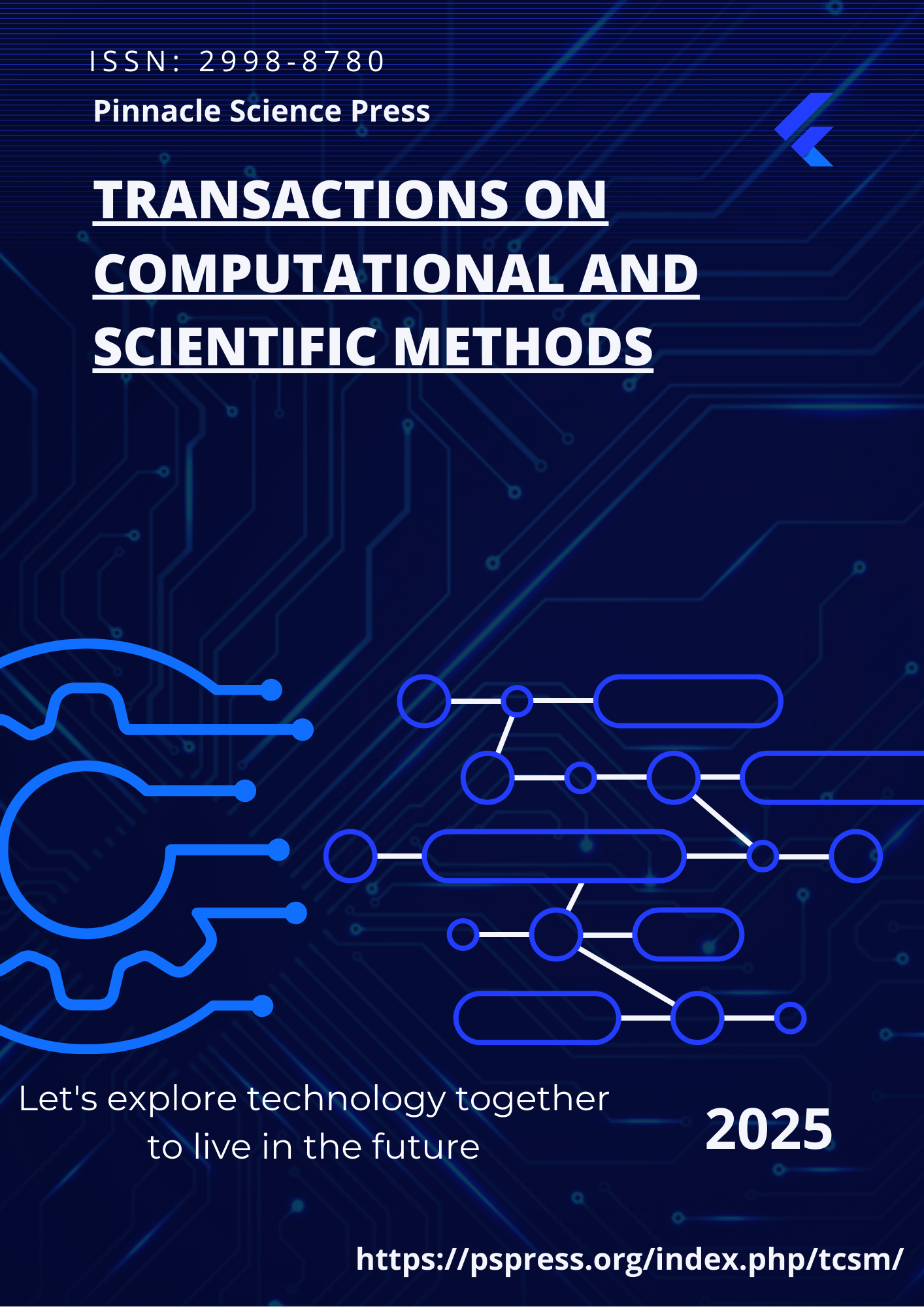
Abstract
This paper studies a natural human-computer interaction system based on gesture key point detection, aiming to achieve accurate interaction between users and virtual devices by efficiently extracting hand key points. The system uses a deep learning model to process gesture images, extract the positions of key points such as finger joints and palms, and convert dynamic gestures into specific instructions through timing analysis. In the simulation experiment, users control virtual devices through gestures and complete remote-control tasks such as light switches, robotic arm operations, and drone path planning. The experimental results show that the system exhibits a high success rate and low latency in static tasks, but still faces certain robustness challenges in dynamic tasks and complex scenarios. Compared with traditional methods, the interaction method based on gesture key points is more natural and intuitive, providing new technical support for application scenarios such as smart homes, industrial automation, and telemedicine. Future research will focus on improving the real-time and adaptability of key point detection, combining multimodal information to further enhance system performance, and expanding its application potential in the fields of virtual reality and augmented reality. The research in this paper not only provides theoretical support for human-computer interaction technology but also lays a foundation for building intelligent control systems in practical applications.