Deep Learning-Driven Advances in Lung Tumor Segmentation and Diagnosis
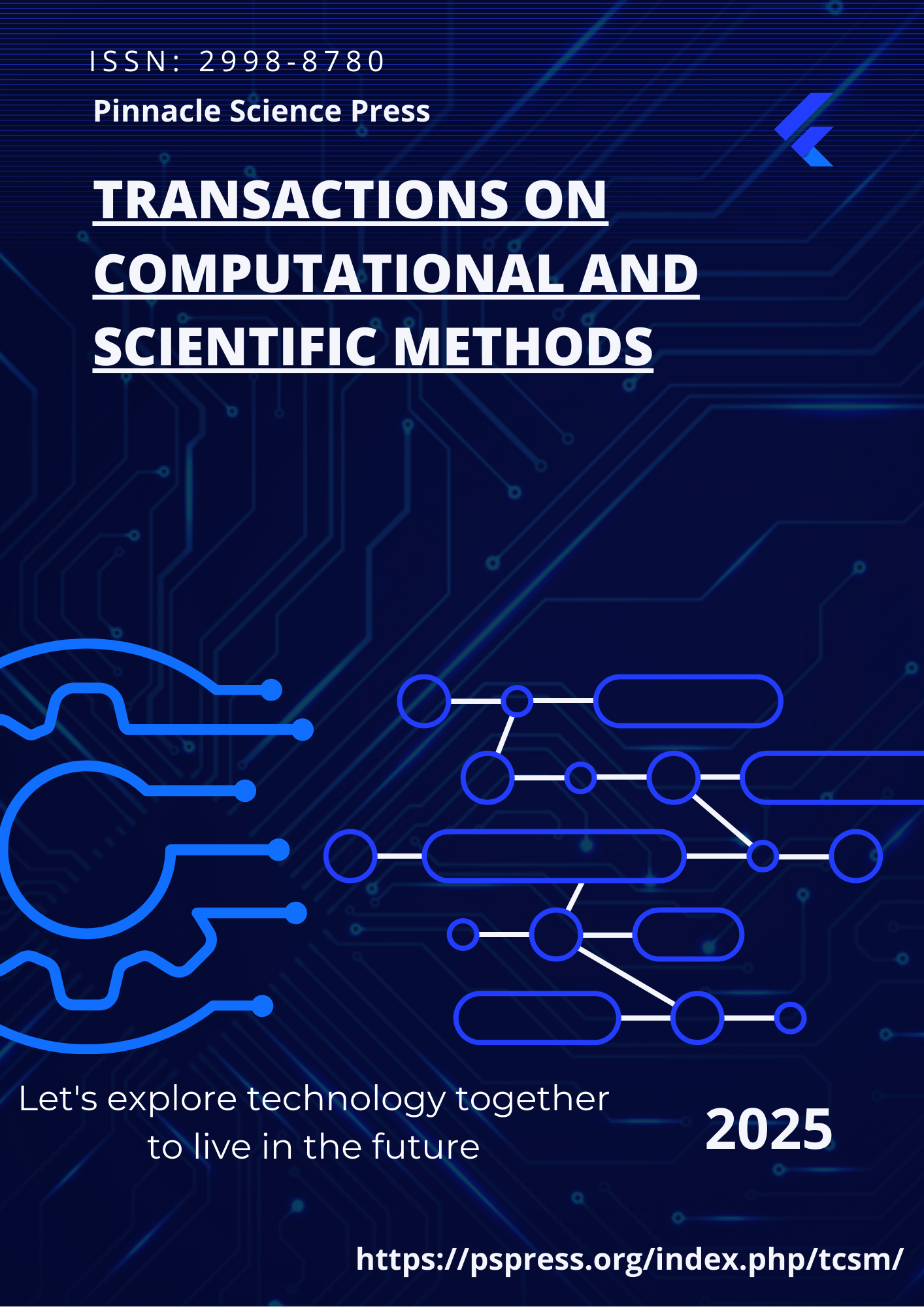
Abstract
Lung tumors, one of the most prevalent respiratory conditions, pose a significant health risk when left undiagnosed and untreated, often progressing to lung cancer with high mortality rates. Traditional imaging screening methods suffer from inefficiencies and frequent diagnostic errors, emphasizing the urgent need for innovative solutions. This study explores the transformative potential of deep learning in enhancing lung tumor diagnosis. By employing the Unet network for CT image segmentation and integrating 3DCNN to filter false positives, the proposed methodology achieves precise lung tumor recognition while minimizing human interference. The findings demonstrate improved diagnostic efficiency and accuracy, providing a robust framework for aiding medical professionals in early detection and reducing diagnostic errors.