Mining Multimodal Data with Sparse Decomposition and Adaptive Weighting
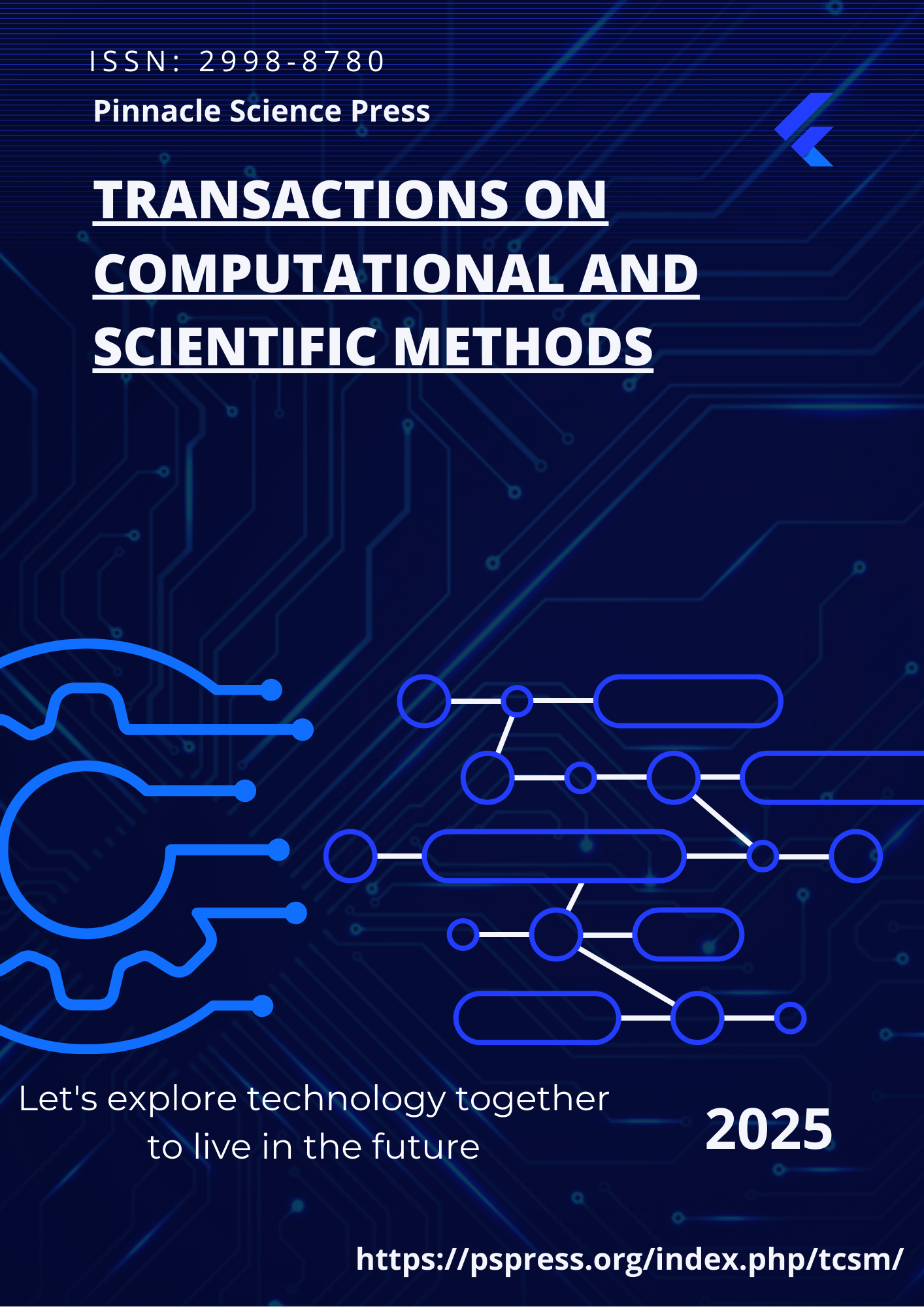
Abstract
This paper proposes a frequent pattern mining method based on multimodal sparse matrix decomposition and dynamic weight optimization to solve the problems of feature imbalance, redundancy, and difficulty in modal fusion existing in multimodal data. Through sparse matrix decomposition technology to extract potential sparse features of multi-modal data, this method can effectively reduce the data dimension and reduce redundant information, while improving the sparsity and expressiveness of feature representation. In addition, the dynamic weight optimization strategy dynamically adjusts the modal weight according to the modal contribution, which enhances the model's adaptability to modal heterogeneity. In experiments, the method in this paper is significantly better than the traditional method in terms of the number of patterns, average support, and operating efficiency, demonstrating its superiority in multi-modal frequent pattern mining. The research results not only provide new technical solutions for multi-modal data mining but also have broad practical application potential. Future research will further optimize the performance of this method on large-scale data and explore its integration with deep learning technology to provide more innovative ideas for complex data analysis and mining.