Performance Boost in Deep Neural Networks: Improved ResNext50 for Complex Image Datasets
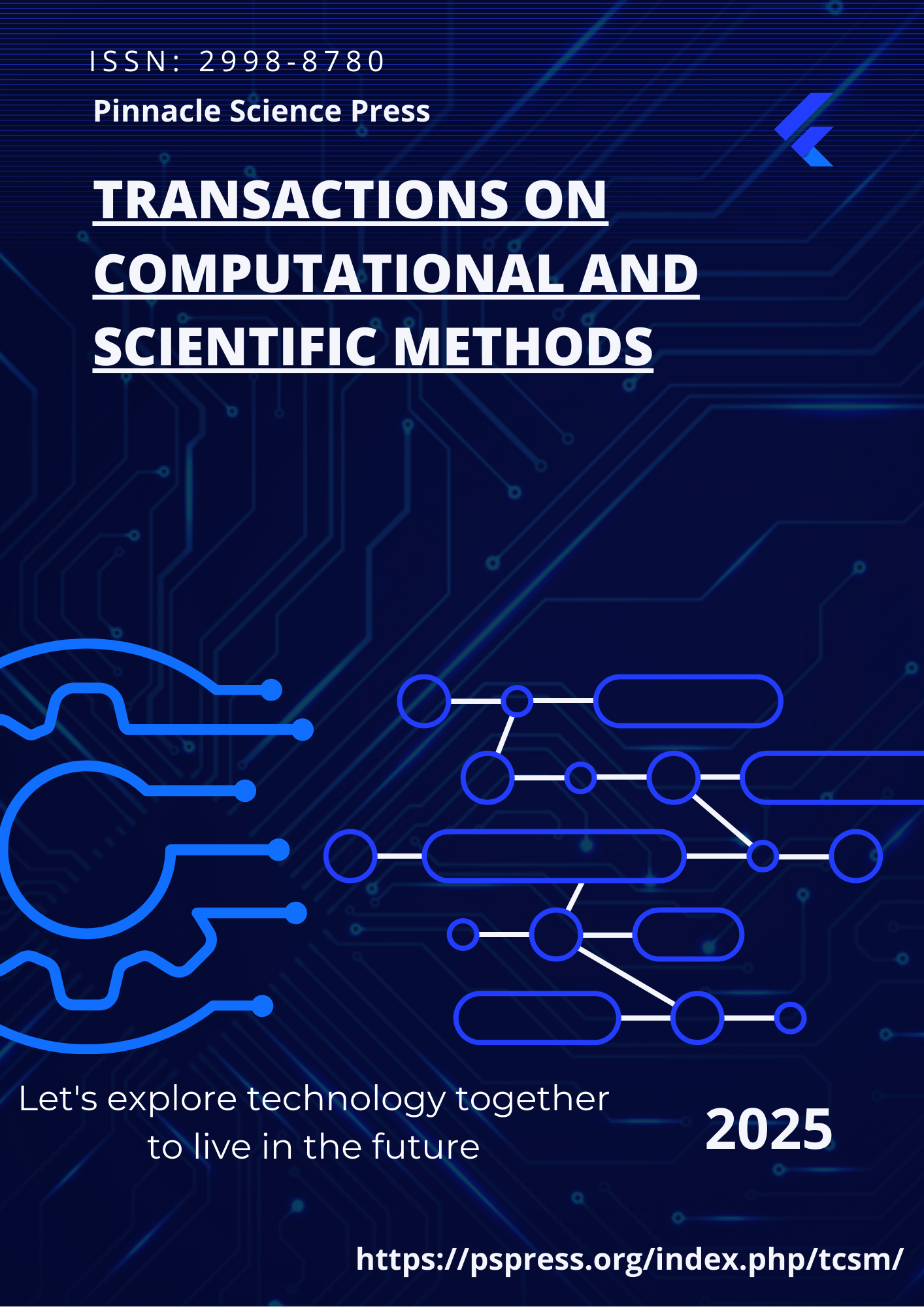
Abstract
This paper proposes an image classification algorithm based on the improved ResNext50, aiming to improve the classification accuracy and robustness of deep neural networks on complex image datasets. By introducing multi-scale feature fusion and adaptive learning rate adjustment, the improved ResNext50 can extract image features more effectively and improve the stability and convergence speed of the network during training. We conducted a large number of experiments on the CIFAR-10 dataset. The results show that compared with VGG, ResNet, Vision Transformer, and the original ResNext, the improved ResNext50 has achieved significant improvements in multiple indicators such as accuracy (ACC), area under the curve (AUC) and F1-Score, showing strong performance and superiority under small samples and noisy images. This model can not only improve the classification accuracy but also has a stronger generalization ability when processing complex image tasks, providing an efficient solution for the field of image classification. Future research can further optimize the model and explore its potential in large-scale datasets and practical applications, especially in the fields of autonomous driving, intelligent monitoring, and medical imaging.