Time-Series Nested Reinforcement Learning for Dynamic Risk Control in Nonlinear Financial Markets
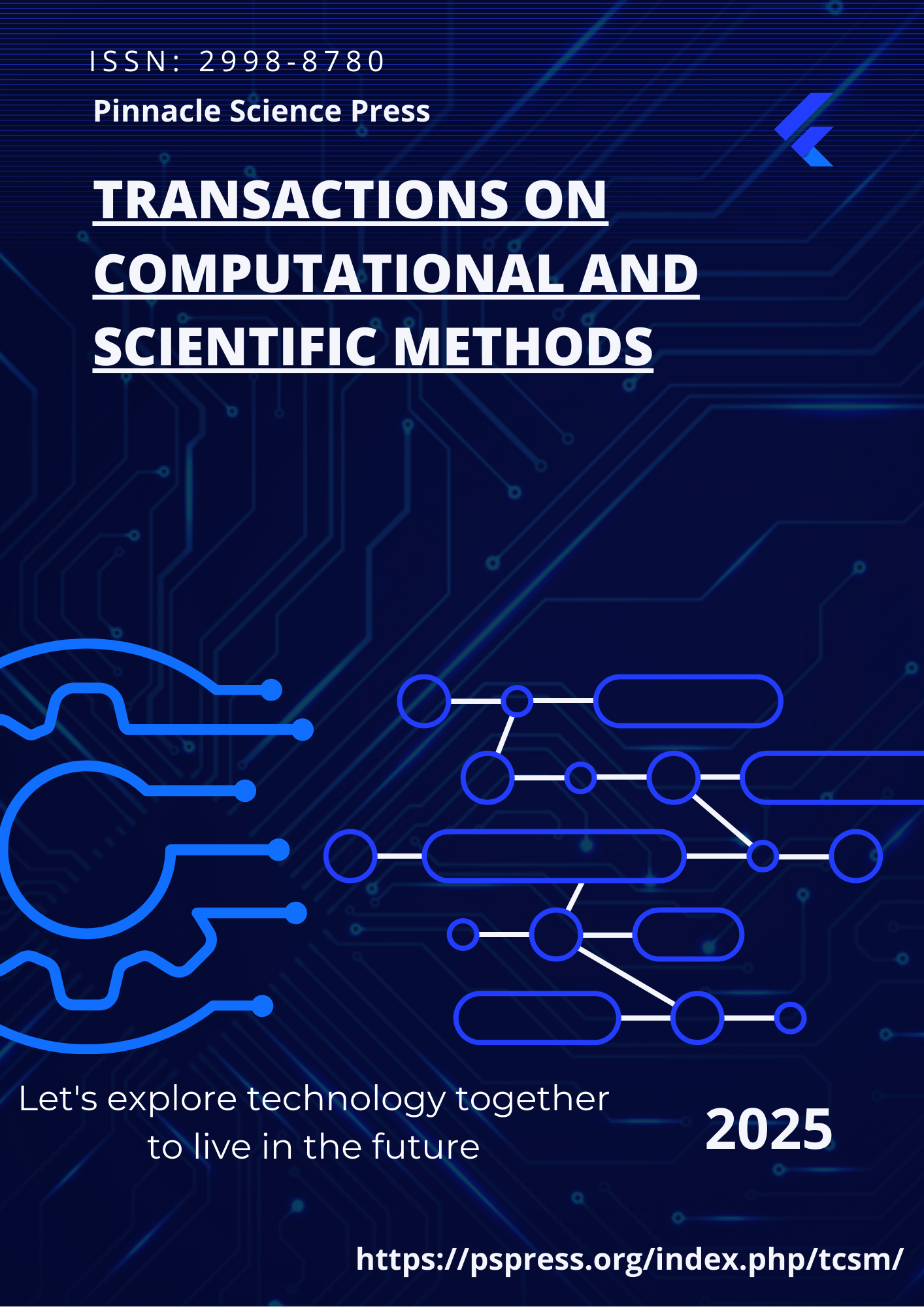
Abstract
This paper proposes a time-series nested reinforcement learning risk control algorithm for nonlinear financial markets, aiming to solve the shortcomings of traditional methods in complex dynamic market environments. By introducing a time series nested structure, the algorithm can jointly model short-term fluctuations and long-term trends, and accurately capture the multi-level dynamic characteristics of the market. At the same time, combined with the multi-objective optimization mechanism, a balance between maximizing returns and minimizing risks is achieved, significantly improving the applicability of risk management and the flexibility of strategies. Experimental results show that the algorithm in this paper performs well in income optimization, risk control, and dynamic adaptability, especially in high-volatility markets and trend reversal scenarios, showing strong robustness and adaptability. Further analysis of the return and risk trade-off curve verified the effectiveness of the multi-objective optimization strategy and provided scientific risk management solutions for different market conditions and investor needs. This research provides a new technical framework for dynamic risk control in complex financial markets and also lays a theoretical foundation for future cross-market and multi-asset portfolio research.