Machine Learning Models for Credit Risk Evaluation and Prediction: Methods and Applications
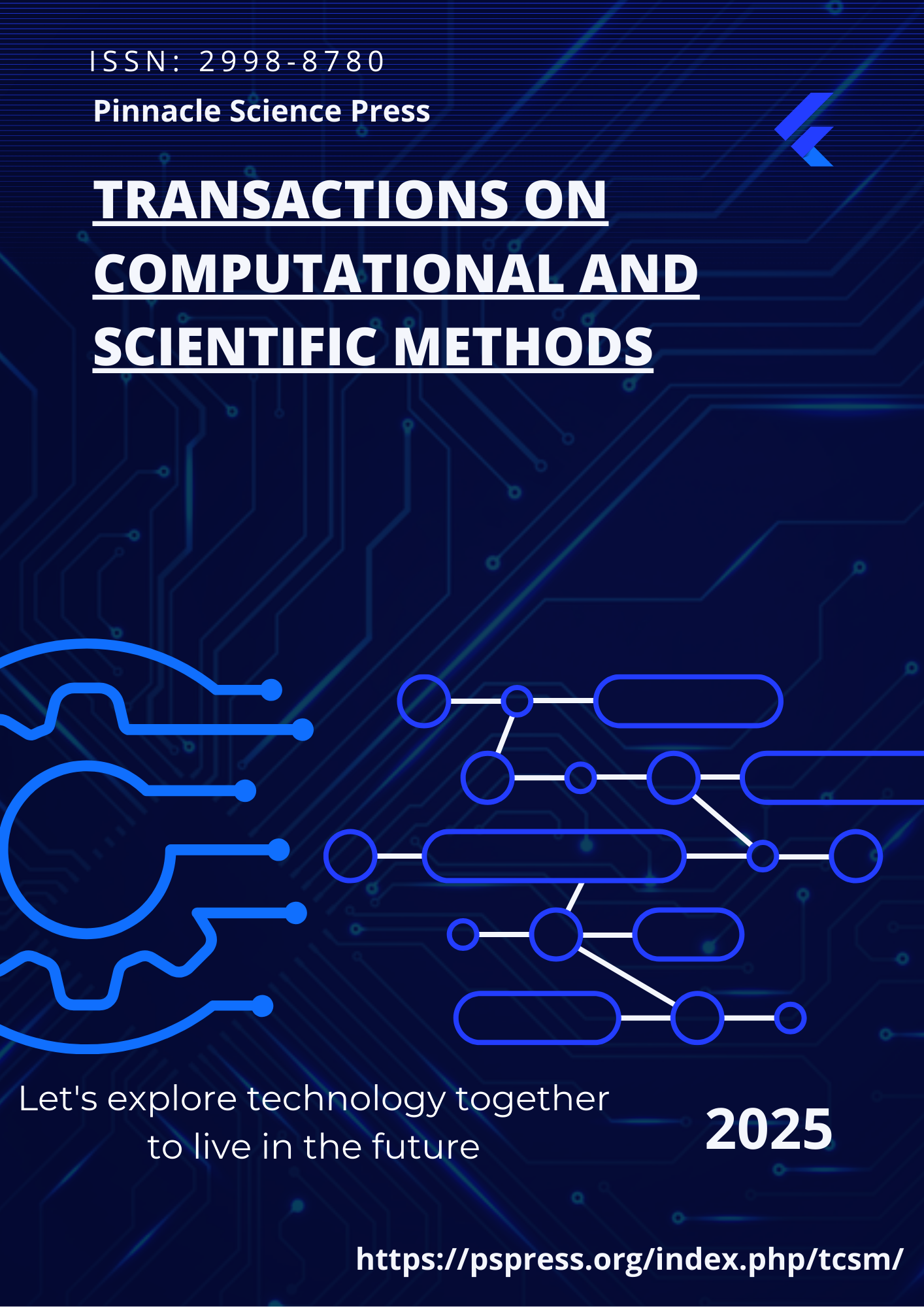
Abstract
The rapid integration of Internet technology and the financial industry, alongside advancements in artificial intelligence and machine learning, has paved the way for innovative risk assessment solutions. Traditional credit risk models, reliant on linear methodologies and subjective expertise, face challenges in addressing the complex, global nature of modern financial risks. This study explores the application of machine learning algorithms-specifically random forests, decision trees, and logistic regression—to predict loan default scenarios. Emphasis is placed on the random forest method for its capability to handle imbalanced datasets and automatically adjust weights based on outcome variables. Experimental results demonstrate the superiority of random forest in classification performance compared to decision trees and logistic regression. Moreover, feature importance analysis identifies key variables, including the borrower's debt ratio, historical overdue instances, and the loan-to-credit ratio, as critical to default prediction. This research contributes to the development of more efficient, data-driven credit risk assessment models and offers insights for feature selection in broader data mining applications.