Audit Fraud Detection via EfficiencyNet with Separable Convolution and Self-Attention
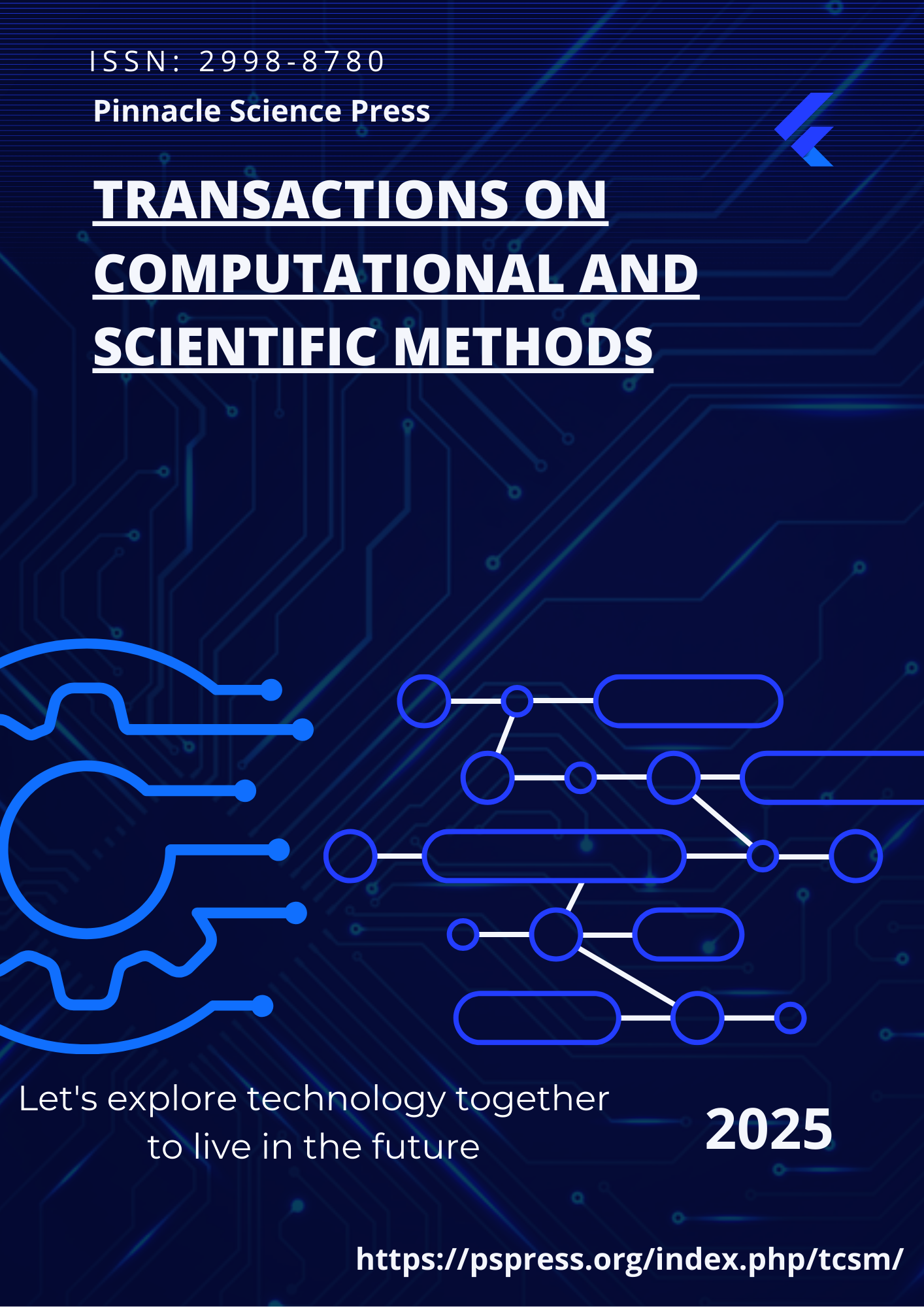
Abstract
This study proposes an audit fraud detection method based on EfficiencyNet, which aims to solve the efficiency and accuracy problems of traditional fraud detection methods in large-scale financial data processing. With the continuous upgrading of financial fraud methods, traditional detection methods based on rule matching and machine learning have limited performance in complex and high-dimensional financial data environments, and it is difficult to accurately capture fraud patterns. To this end, this study introduces Depthwise Separable Convolution to reduce computational costs and combines the self-attention mechanism to enhance the model's learning ability for key features, thereby improving the recognition accuracy of fraudulent behavior. The experiment uses a data set containing multi-dimensional financial indicators to compare the detection capabilities of traditional machine learning and deep learning models, such as logistic regression (LR), random forest (RF), XGBoost, LSTM, and CNN. Experimental results show that the EfficiencyNet model performs well in key indicators such as AUC, F1-score, Precision, and Recall, especially the EfficiencyNet B3 variant, which has an AUC of 0.96 and an F1-score of 0.91, far exceeding traditional methods. In addition, the impact of different components on model performance was further analyzed through ablation experiments. The results show that deep separable convolution and self-attention mechanisms play a key role in improving the detection ability of the model. In terms of data processing, this study adopts data enhancement, category balancing strategy, and cross-validation to ensure that the model can still maintain strong generalization ability when fraud data is scarce. In the future, as financial fraud methods become more complicated, research can further introduce adaptive learning, time series modeling, and explainable AI technology to improve the dynamic adaptability and credibility of fraud detection. In addition, this research method can be extended to the fields of blockchain auditing, supply chain finance, and financial risk control, providing enterprises and regulators with intelligent fraud detection solutions, thereby building a more transparent and secure financial system.