Efficient Market Signal Prediction for Blockchain HFT with Temporal Convolutional Networks
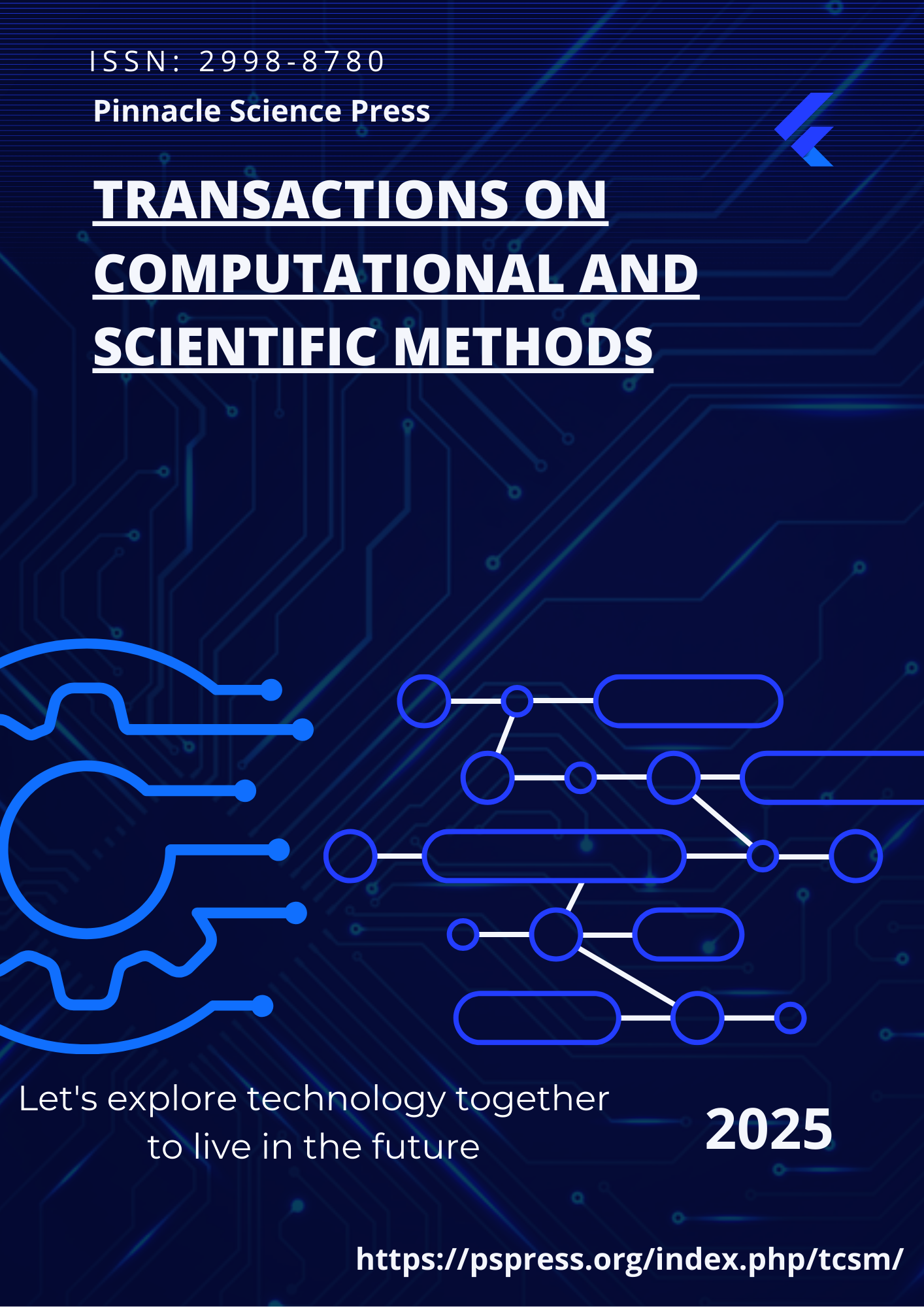
Abstract
High-frequency trading in the blockchain market has extremely high time sensitivity and complex market dynamics. Traditional time series modeling methods have problems with low computational efficiency and difficulty in capturing long-term dependencies in trading signal prediction. To address this challenge, this study proposes a trading strategy optimization method based on temporal convolutional networks (TCNs). TCN improves the modeling ability of market dynamics through causal convolution and dilated convolution mechanisms while ensuring efficient parallel computing capabilities. Experimental results show that this method is superior to traditional models in terms of prediction accuracy, cumulative yield, and risk control and significantly improves the stability of trading strategies. In addition, the contribution of TCN key components to trading strategy optimization is analyzed through ablation experiments, verifying the importance of dilated convolution and residual connections in improving the ability to predict trading signals. This study provides an efficient and accurate intelligent optimization solution for blockchain high-frequency trading and provides new ideas for the strategy design of future decentralized trading markets.