Advancements and Challenges in Image Recognition: A Comparative Analysis of Traditional Neural Networks and Convolutional Neural Networks
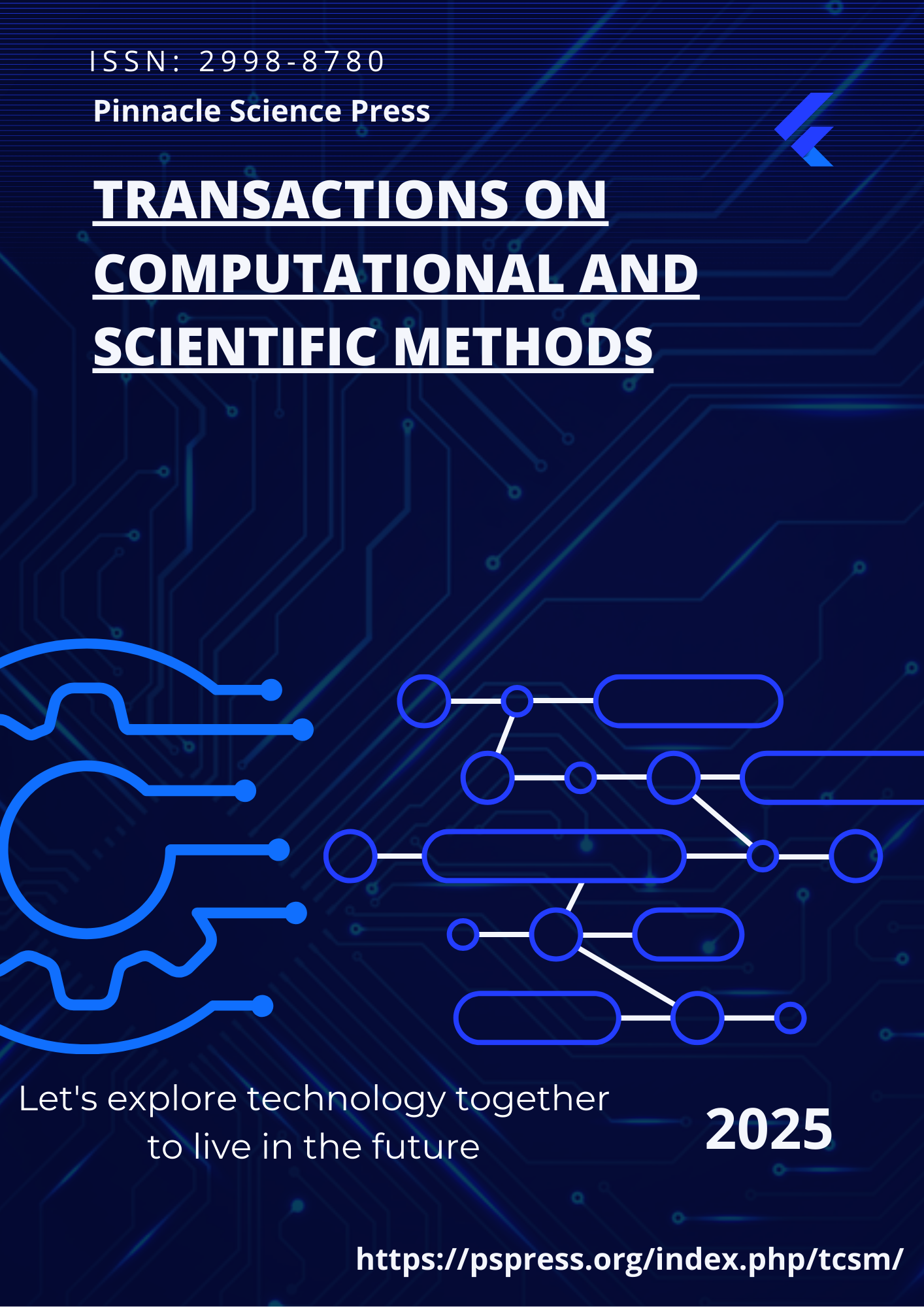
Abstract
Image recognition technology has evolved significantly since its inception in the 1940s, with notable advancements occurring in the 1990s through the integration of artificial neural networks and support vector machines. While traditional image recognition methods relied on shallow hierarchical structures and manual preprocessing, neural networks have revolutionized the field by enhancing accuracy and adaptability. This paper analyzes the advantages and limitations of both traditional neural networks and convolutional neural networks (CNNs), exploring their principles and real-world applications. With the increasing complexity of image recognition environments, standalone CNN techniques face challenges such as color similarity between objects and backgrounds, overlapping targets, and extreme occlusion. To address these limitations, integrating multiple CNN models and designing specialized neural network structures for different object types can further enhance recognition accuracy.