Development of a Deep Learning-Enhanced Chemiluminescence Method for Trace Formaldehyde Detection in Water Samples
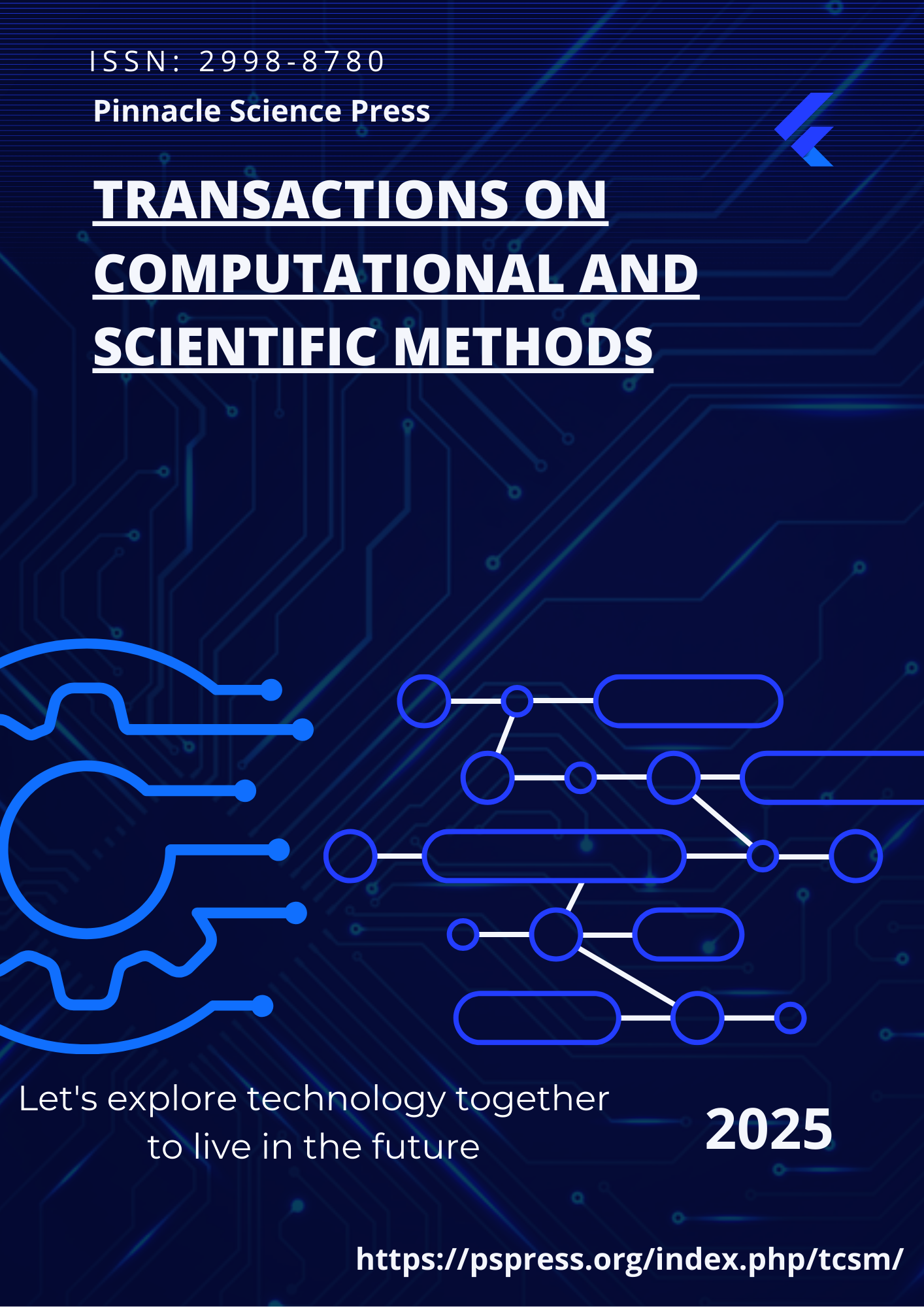
Abstract
Formaldehyde, a widely encountered organic pollutant and recognized Group I carcinogen, poses significant threats to human health even at trace concentrations. This study presents a chemiluminescence-based method for the sensitive detection of trace formaldehyde in water, enhanced by deep learning algorithms for signal processing and concentration prediction. The detection system utilizes an optimized gallic acid reagent system, combined with a photomultiplier tube (PMT) for signal acquisition. Chemiluminescence signals are processed using a convolutional neural network (CNN) to denoise, extract features, and predict formaldehyde concentrations in real time. The method achieves a detection limit of 5.96×10⁻⁵ μg/mL, outperforming conventional phenol reagent methods in terms of simplicity, speed, sensitivity, and accuracy. Its capability for online and portable detection makes it a promising approach for real-time water quality monitoring and environmental analysis.