Time-Series Premium Risk Prediction via Bidirectional Transformer
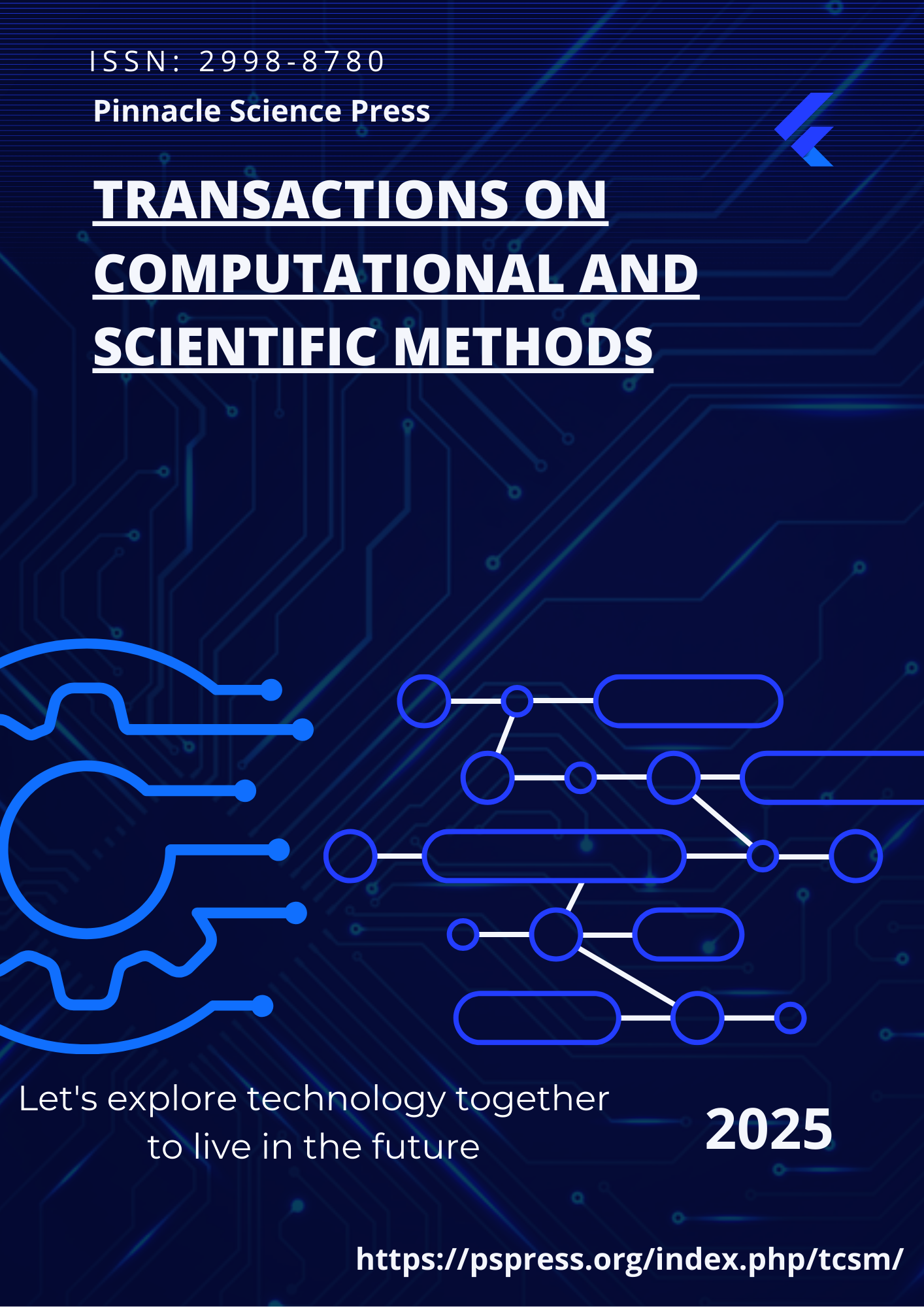
Abstract
This paper proposes a financial premium risk prediction model based on a bidirectional Transformer, aiming to enhance the prediction accuracy of premium risk in the financial market. With the increasing uncertainty and complexity of financial markets, traditional risk prediction methods often fail to effectively address the challenges posed by nonlinear features and high-dimensional data. As a result, deep learning-based prediction models have garnered increasing attention. The bidirectional Transformer, which can simultaneously process past and future information, excels in modeling time series data and is particularly well-suited for handling complex financial data. By incorporating the self-attention mechanism and a bidirectional structure, this study is able to fully capture the nonlinear relationships and temporal dependencies inherent in financial markets, thereby providing a more accurate model for premium risk prediction. In the experimental section, this paper compares the proposed model with traditional regression models, neural network models, and other deep learning approaches. The results demonstrate that the proposed model outperforms the comparison models across multiple evaluation metrics (e.g., mean squared error, mean absolute error, and R²), validating its effectiveness in predicting financial premium risk. To further assess the contribution of key components to the model's performance, an ablation study was conducted. The findings indicate that the self-attention mechanism and the bidirectional Transformer structure are the critical factors driving the model's performance improvements. Overall, the experimental results suggest that the proposed model is better equipped to adapt to the complex fluctuations of the financial market, offering a novel approach to financial risk prediction. Future research may focus on enhancing the model's prediction accuracy and generalization ability by incorporating additional data sources and advanced techniques, such as neural networks, to provide more efficient risk management tools for the financial industry.