Research on Tumor Classification and Detection Algorithms Based on CNN
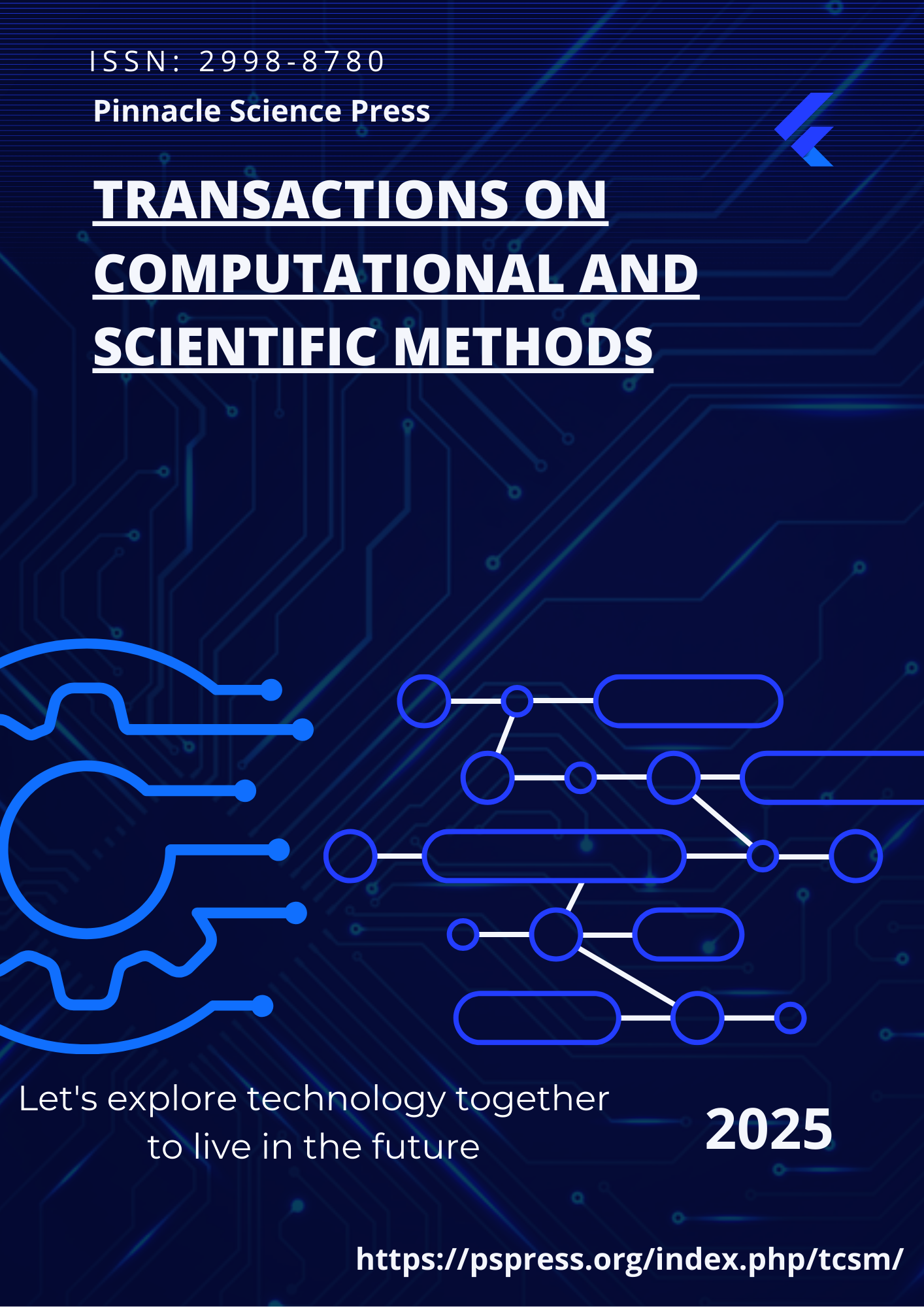
Abstract
This study focuses on the use of Convolutional Neural Networks (CNNs) for tumor classification and detection. Several machine learning models, including Support Vector Machine (SVM), Random Forest (RF), Long Short-Term Memory (LSTM), Decision Tree (DT), and Multi-Layer Perceptron (MLP), were compared to evaluate their performance in medical image analysis. The experimental results show that CNN outperforms all other models, achieving the highest accuracy and recall rates. CNN’s ability to automatically extract complex spatial features from medical images makes it particularly suitable for tasks such as tumor classification, where precision and sensitivity are crucial. While other models such as SVM and RF also exhibited decent performance, they were unable to match the effectiveness of CNN in processing high-dimensional, complex image data. The Decision Tree model, though easy to interpret, showed the weakest performance in this task. These findings underscore the superiority of CNN in medical image classification and suggest that further optimization of CNN architectures could lead to even more effective tumor detection methods.