Credit Card Fraud Detection via Hierarchical Multi-Source Data Fusion and Dropout Regularization
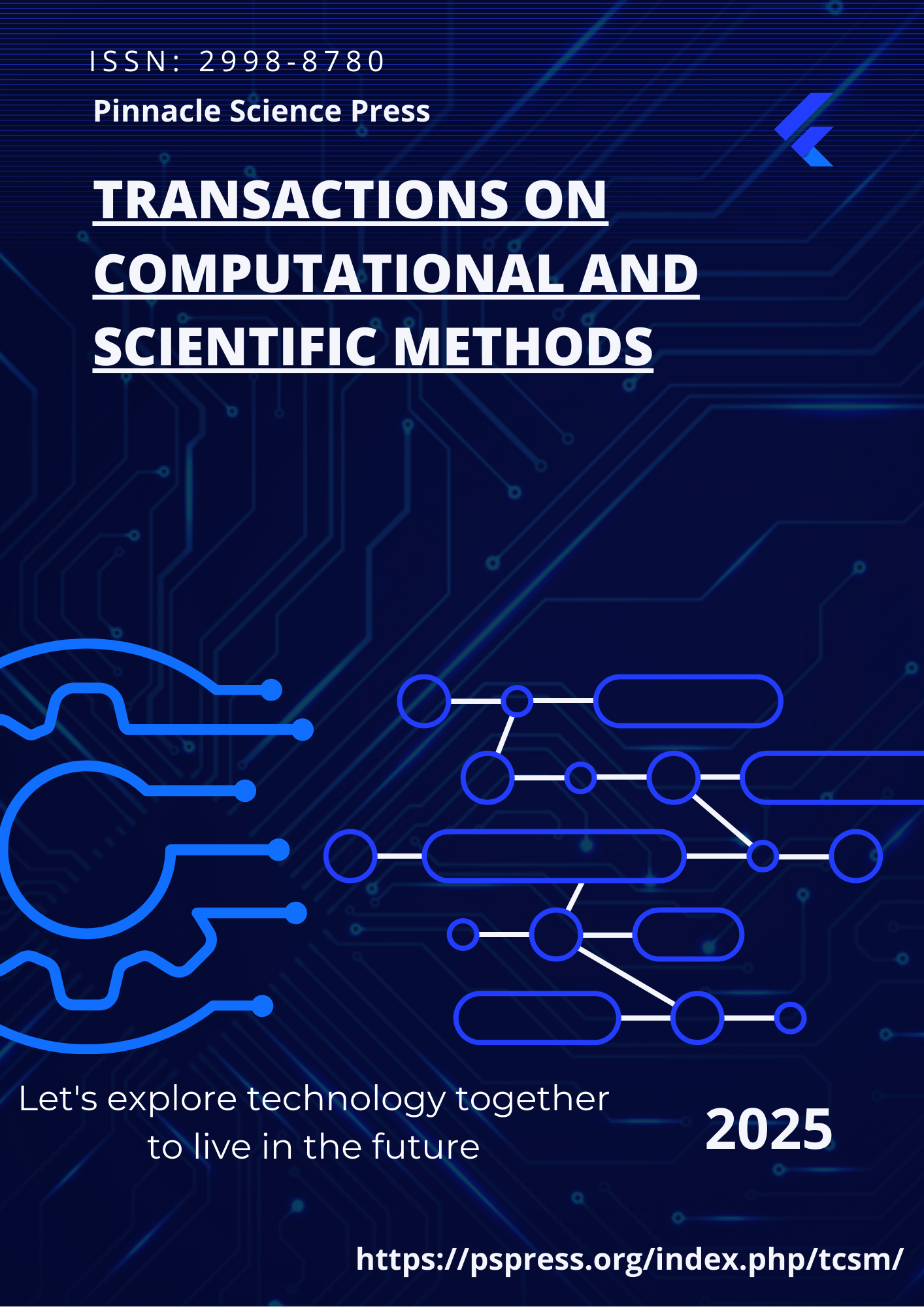
Abstract
This paper proposes a credit card anomaly detection algorithm based on hierarchical multi-source data feature fusion, aiming to improve the accuracy and efficiency of traditional methods in identifying fraud in the financial field. By combining multiple source data features such as transaction time, amount, location, and user historical behavior, the model can fully capture potential abnormal patterns in transaction data. In addition, the hierarchical feature fusion strategy and Dropout regularization technology are adopted to effectively improve the robustness and generalization ability of the model. Experimental results show that the proposed model exceeds various traditional methods and deep learning models such as XGBoost, RNN, decision tree, random forest, and MLP in terms of evaluation indicators such as accuracy, AUC, and F1-Score. Further ablation experiments verify the important role of multi-source data fusion, hierarchical fusion, and Dropout regularization in improving model performance. Although relatively ideal results have been achieved, this study still faces challenges such as data privacy protection and real-time computational efficiency. In the future, unsupervised learning, semi-supervised learning, and efficient computing methods can be further explored to achieve wider application and promotion in the field of financial fraud detection.