Enhanced SEIARN Infectious Disease Modeling with Adaptive Parameter Estimation Using Sparrow Search Algorithm
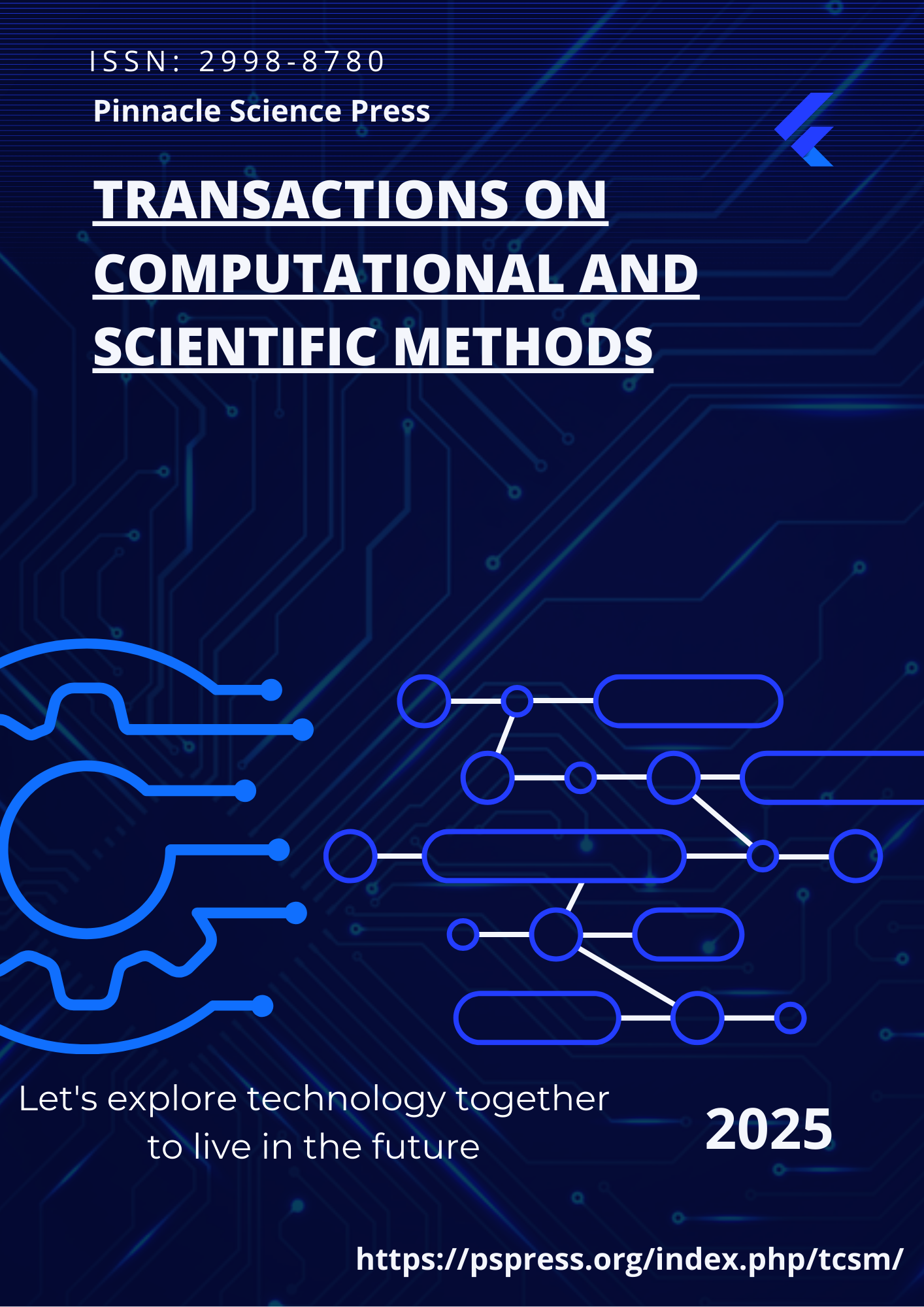
Abstract
Infectious diseases pose a significant threat to global public health, impacting both human well-being and socio-economic development. The SEIARN model is a widely used epidemiological framework for predicting disease transmission by categorizing individuals into different states. However, accurate parameter estimation remains a major challenge, as traditional methods relying on empirical and statistical approaches often suffer from limitations in precision and stability. To address this issue, this paper proposes an adaptive parameter estimation model, SSA-SEIARN, which leverages the Sparrow Search Algorithm (SSA) to dynamically optimize SEIARN parameters.Compared to conventional approaches, SSA-SEIARN enhances model accuracy and stability by adaptively adjusting key parameters, including the latency infection rate (a ), infection recovery rates ( g1 and g 2 ), and the probability of secondary infection (q ). Simulation experiments demonstrate that SSA-SEIARN significantly improves the fitting performance of the model, making it a more reliable tool for infectious disease prediction. The findings of this study provide valuable insights into the application of optimization algorithms in epidemiological modeling and offer a novel approach to refining disease transmission predictions.