Single-Device Human Activity Recognition Based on Spatiotemporal Feature Learning Networks
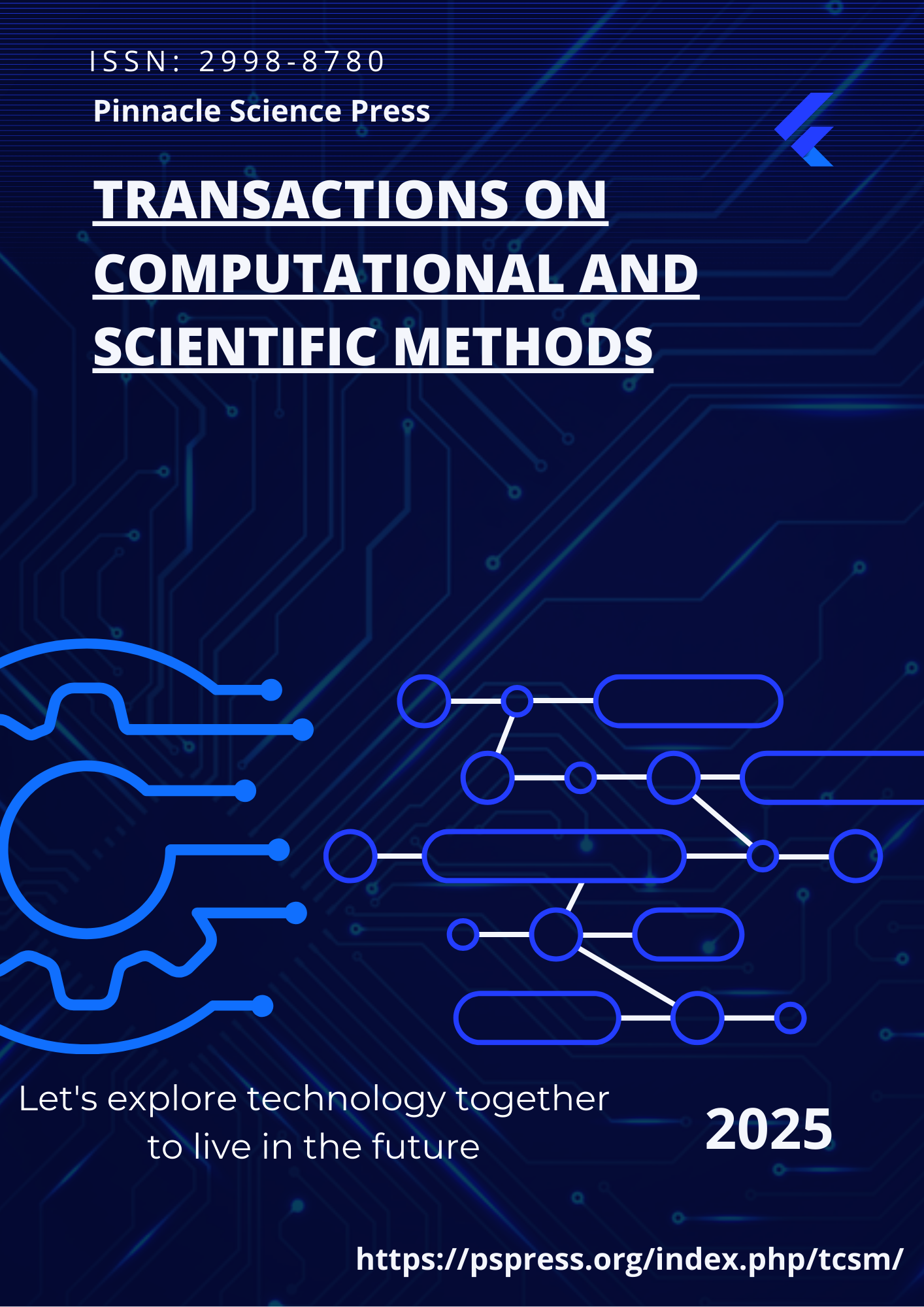
Abstract
This study proposes a single-source device human behavior recognition method based on a dual-branch spatiotemporal feature extraction network to solve the problem of limited modeling capabilities of traditional methods in single-sensor data. This method combines the spatiotemporal convolution branch and the temporal modeling branch to extract local motion patterns and capture long-term temporal dependencies, respectively, so as to achieve accurate classification of different human behaviors without relying on multi-device data fusion. The experiment was verified on the WISDM dataset and compared with mainstream methods such as 3DCNN, LSTM, RNN and Transformer. The results show that the model proposed in this study has achieved the best performance in multiple indicators such as accuracy (ACC), F1 value, Precision and Recall, and significantly improved the accuracy and robustness of single-source device behavior recognition. Although this study has achieved good results in performance optimization, there is still a problem of high computational complexity. In the future, we can explore a lighter network structure and combine multimodal data fusion and self-supervised learning to further improve the generalization ability and adaptability of the model. The results of this study can be widely used in fields such as intelligent health monitoring, motion analysis, intelligent security, and rehabilitation training, providing new technical support for human behavior recognition of wearable devices.