MS-UNet: A Transformer-Based Multi-Scale Nested Decoder Network for Medical Image Segmentation with Limited Data
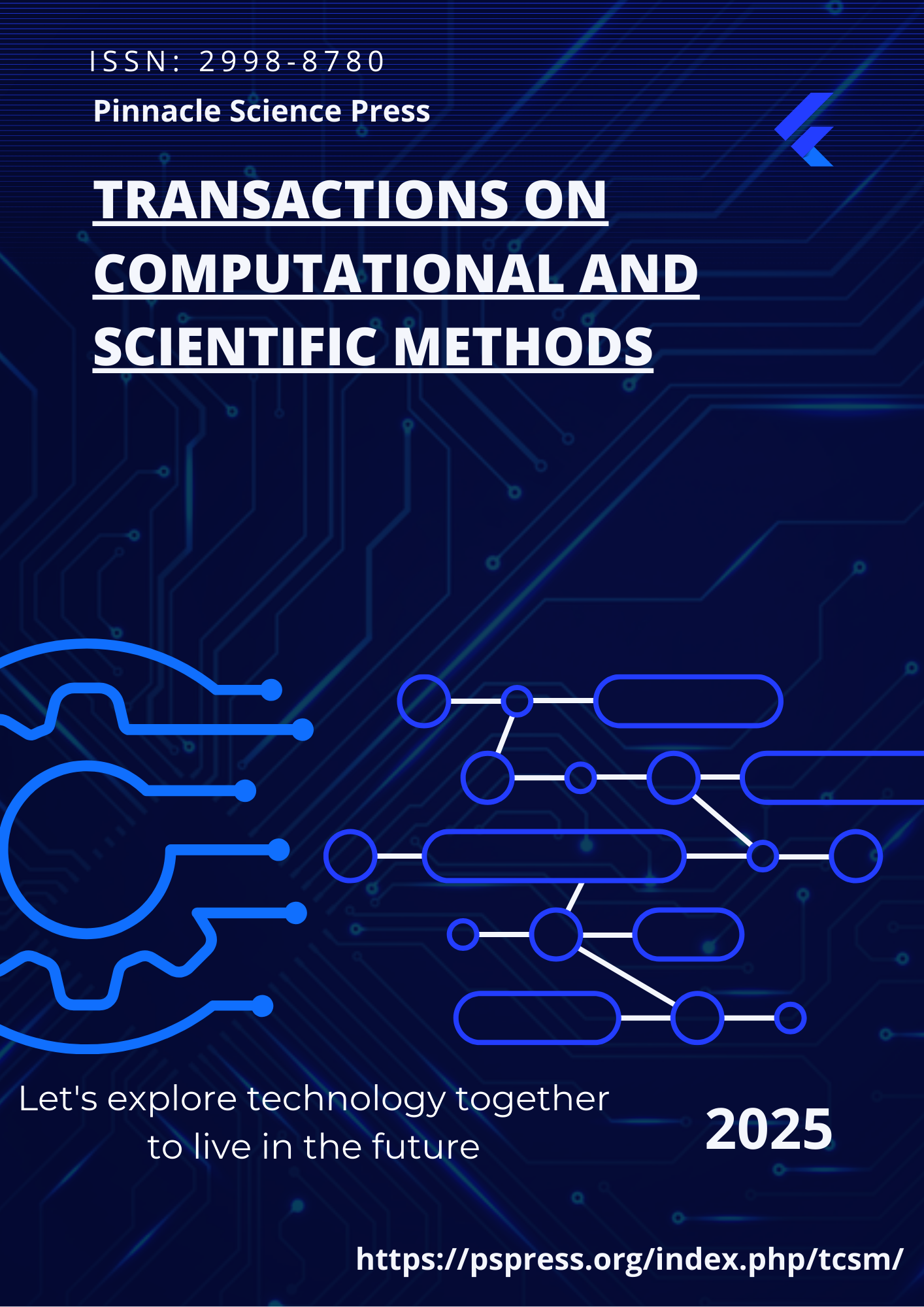
Abstract
With the rapid advancement of deep learning, neural networks have demonstrated remarkable progress in medical image segmentation, significantly enhancing the accuracy and efficiency of lesion detection and organ boundary identification. Traditional medical image segmentation relied on manually designed features, which were labor-intensive and struggled with complex image variations. The emergence of Convolutional Neural Networks (CNNs), particularly UNet and its variants, revolutionized the field by leveraging hierarchical feature extraction. More recently, inspired by breakthroughs in Natural Language Processing (NLP), Transformer-based models, such as Vision Transformer (ViT) and Swin Transformer, have been successfully applied to medical image segmentation, addressing CNNs' limitations in capturing long-range dependencies.However, the direct application of Transformer models introduces challenges, such as a semantic gap between the encoder and decoder, which can hinder segmentation performance. To address this, we propose MS-UNet, a Transformer-based multi-scale nested decoder segmentation framework that enhances feature learning and semantic communication between network modules. By designing a dense multi-scale nested decoder, MS-UNet effectively mitigates the semantic discrepancy, improving segmentation accuracy, especially in scenarios with limited training data. Experimental results on MRI and CT segmentation tasks demonstrate that MS-UNet significantly outperforms CNN-based models and other Transformer-based architectures. This study not only provides an effective solution to medical image segmentation under data-scarce conditions but also offers a novel approach for broader applications in medical imaging.