Channel Pruning for Lightweight UNet in Medical Image Segmentation
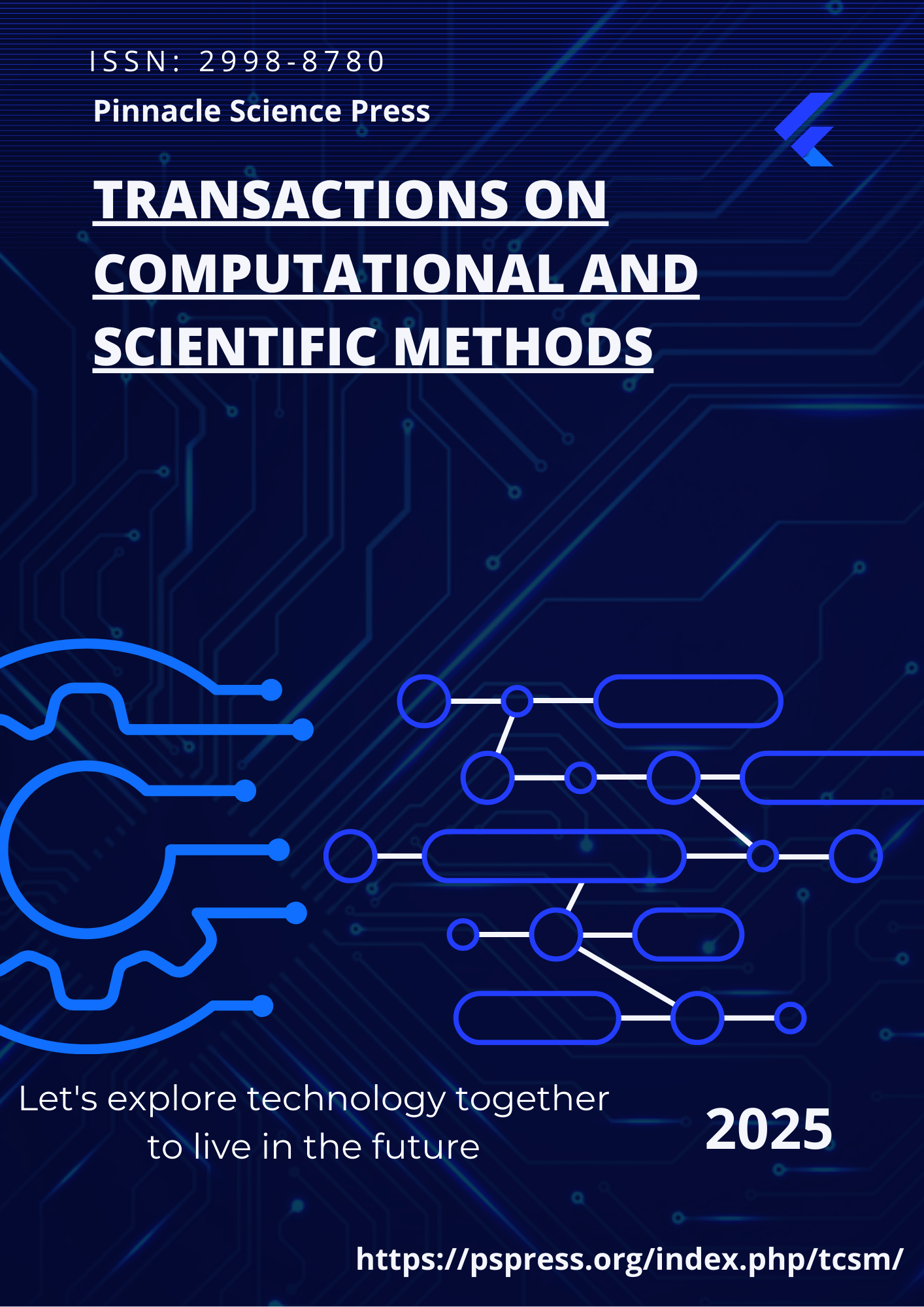
Abstract
UNet has become a cornerstone in medical image segmentation due to its strong performance, yet its high computational complexity poses challenges for real-world deployment. This paper presents a structured channel pruning method that removes less important convolutional channels to reduce model size and accelerate inference. The approach maintains the original architecture while significantly improving computational efficiency, enabling real-time performance on edge devices and in clinical settings with limited resources. Experiments on benchmark datasets, including ISIC 2018 and BraTS, demonstrate that the pruned UNet achieves substantial reductions in parameters and inference time with minimal impact on segmentation accuracy. This work offers a practical solution for compressing UNet models without redesigning the network or relying on specialized hardware.