Sequential Recommendation via Time-Aware and Multi-Channel Convolutional User Modeling
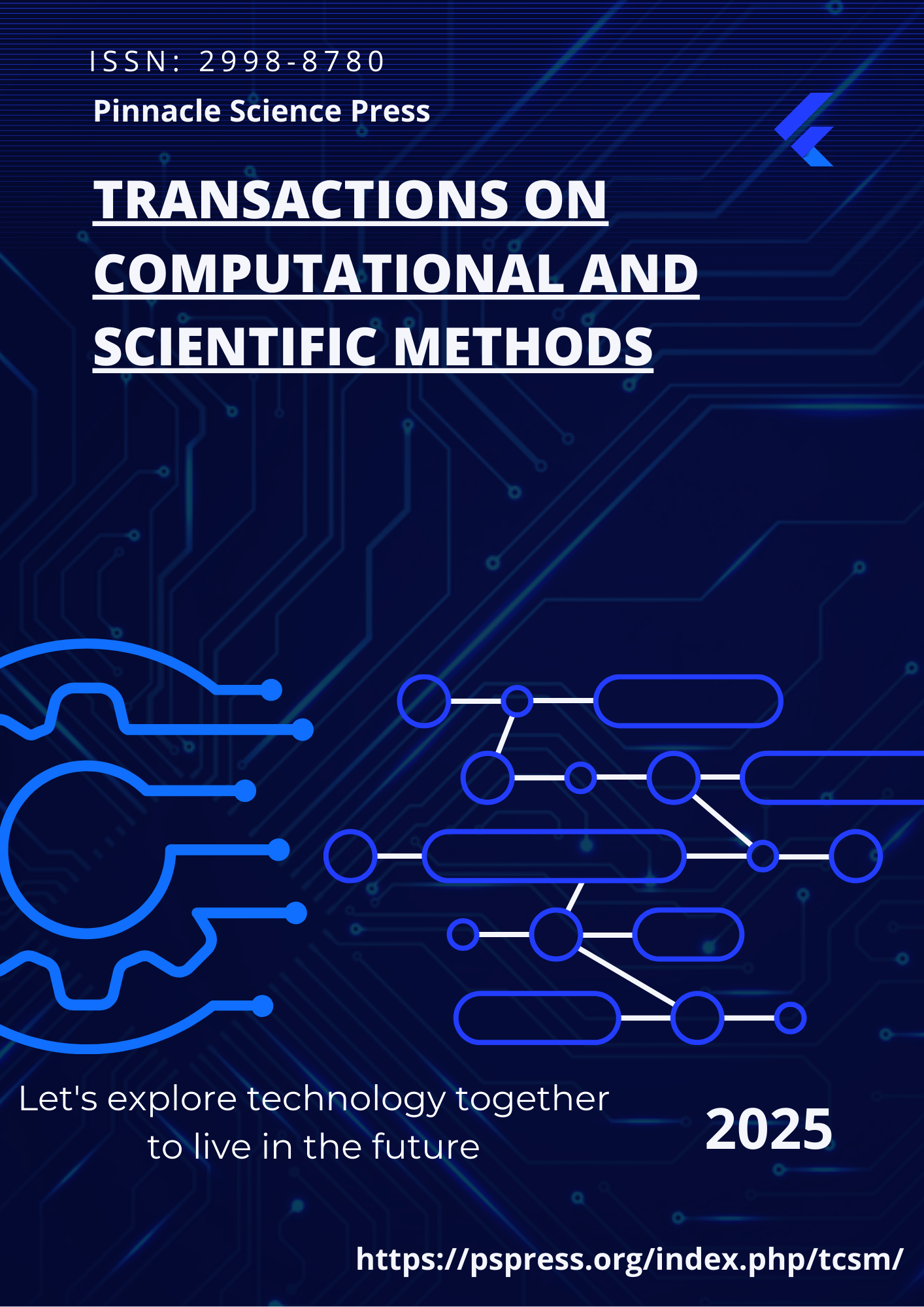
Abstract
This paper proposes a 1D-CNN-based sequential recommendation algorithm that integrates temporal awareness and multi-interest modeling to enhance the understanding of dynamic user behaviors. The model introduces time interval encoding and a multi-channel convolutional structure to effectively capture both short-term and long-term user preferences. Unlike traditional methods that rely on static profiles or single-vector representations, the proposed approach enables more fine-grained modeling of user interest evolution within sequential data. Extensive experiments are conducted on a real-world dataset, covering multiple evaluation metrics and testing scenarios, including varying sequence lengths, user activity levels, and convolutional configurations. The results demonstrate that the proposed method consistently outperforms several state-of-the-art baselines in recommendation accuracy, robustness, and generalization ability. This research provides a lightweight and efficient solution for large-scale recommender systems, contributing to the advancement of sequential modeling techniques and reinforcing the role of temporal and interest-aware strategies in personalized recommendation tasks.