A Predictive Model for NOx Emissions in Coal-Fired Boilers Based on PCA-MFOA-LSSVM Integration Method
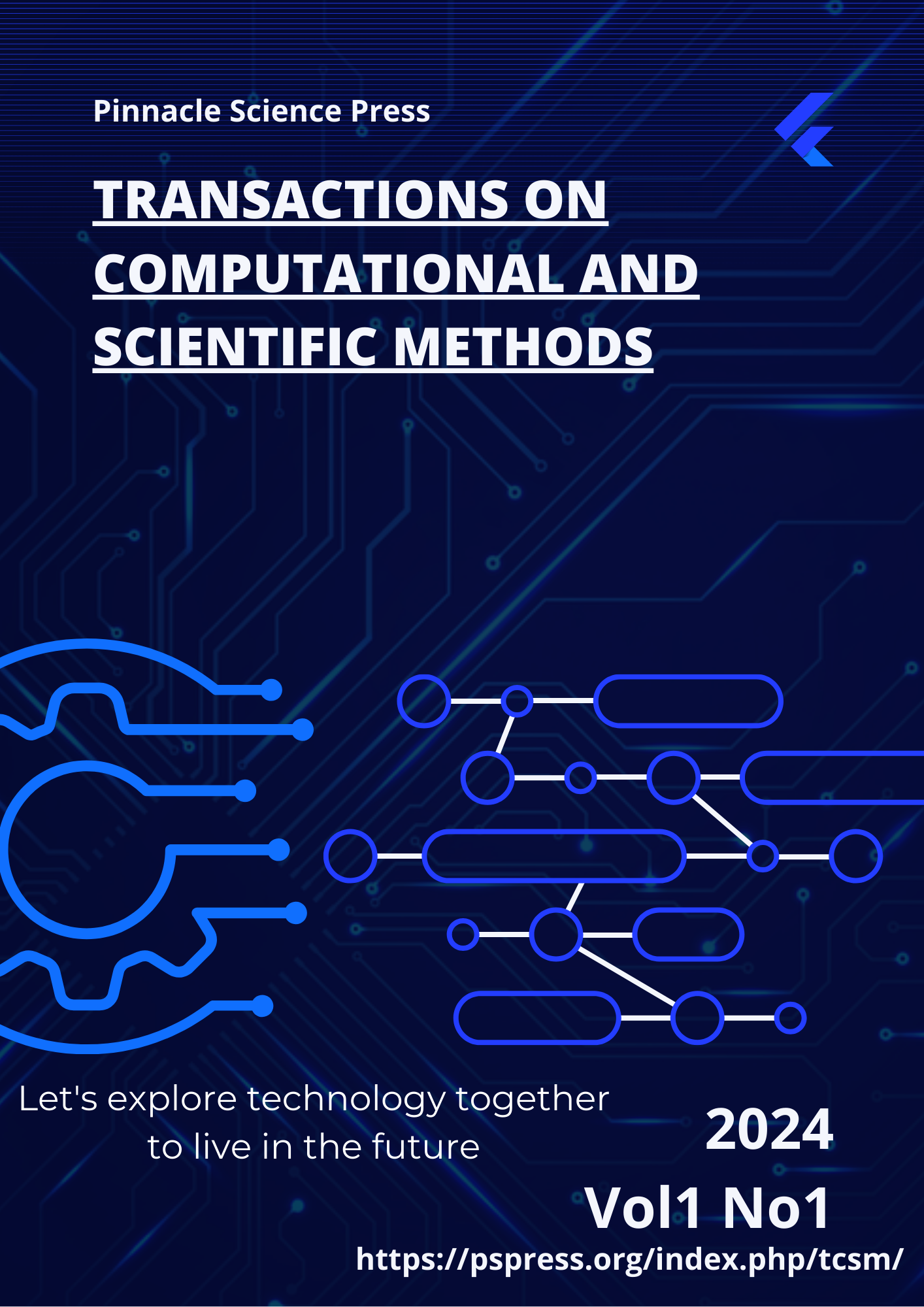
Abstract
The long prediction cycle and low accuracy of NOx emissions forecasts from power plant boilers significantly hinder effective pollutant control and emission reduction efforts. To address this, a predictive method integrating Principal Component Analysis (PCA), Modified Fruit Fly Optimization Algorithm (MFOA), and Least Square Support Vector Machine (LSSVM) is proposed. Initially, the high-dimensional sample matrix undergoes preprocessing. The sample space is then segmented based on the normalized levels of NOx emissions. PCA is employed to extract principal components from each subspace, thereby reducing dimensionality. The FOA is adapted into MFOA by incorporating an adaptive step size and modifying the odor determination value. Subsequently, LSSVM is utilized to develop prediction models for each subspace, with the kernel parameter and penalty factor optimized globally using MFOA. Finally, these sub-models are integrated through segmentation fitting to generate the overall model output. Simulation results demonstrate that the PCA-MFOA-LSSVM integrated method enhances prediction accuracy and reduces prediction time compared to other prediction models.