Enhanced Image Fusion Using Shearlet Transform and Pulse-Coupled Neural Networks
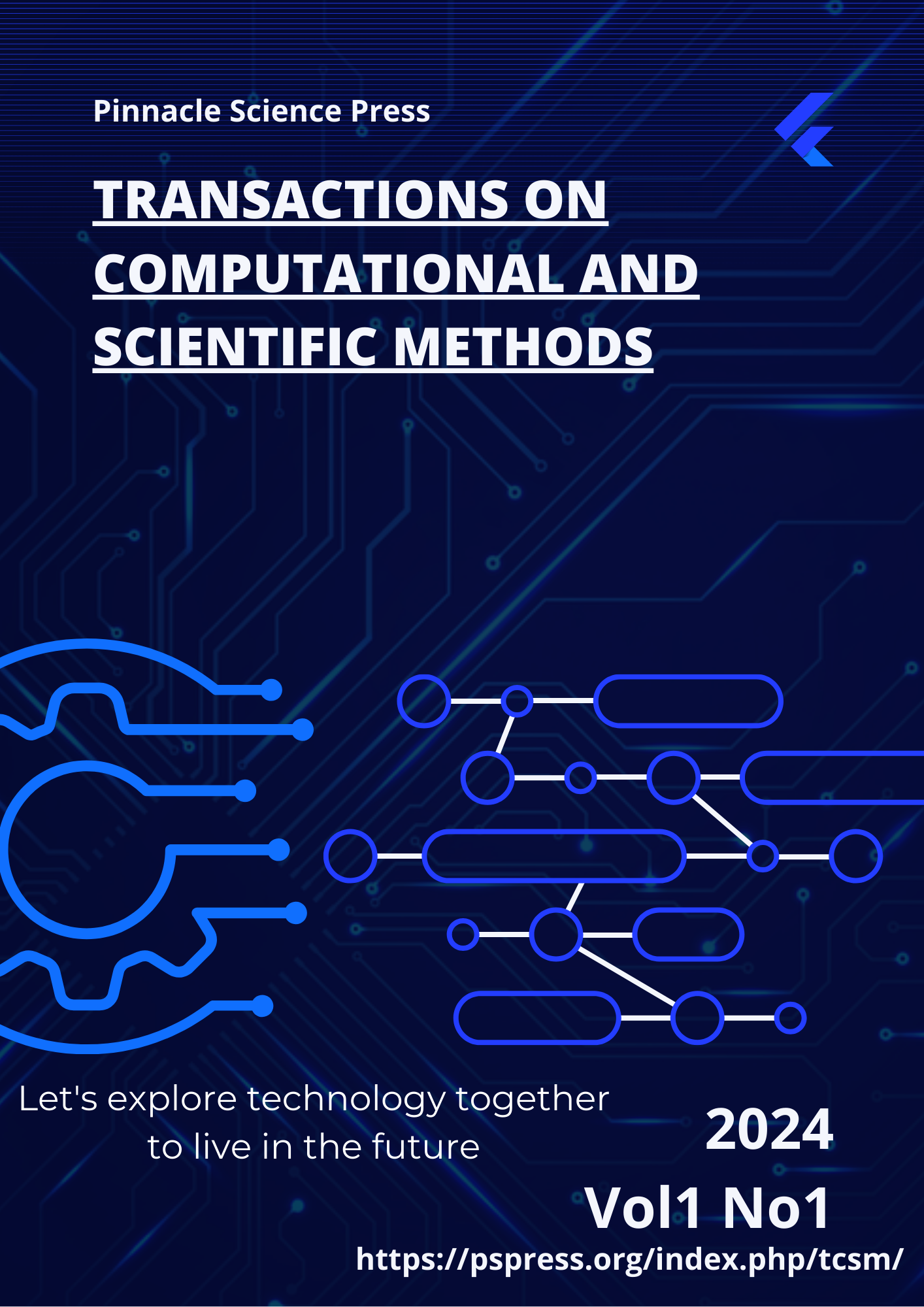
Abstract
Traditional multi-scale image fusion techniques often struggle to maintain translation invariance during the multi-directional and multi-scale decomposition of images. While the non-downsampled contourlet transform (NSCT) exhibits multi-scale, multi-directional, and translation-invariant properties, it is limited by directional constraints. This paper introduces a novel image fusion framework based on the non-subsampled shearlet transform (NSST) domain. This framework effectively preserves image energy and detail while addressing the directional limitations in image decomposition. The proposed method employs NSST for decomposing the source images and utilizes a pulse coupled neural network (PCNN) model to differentiate the absolute values of high-frequency coefficients across various source images. The low-frequency fusion rule is derived from low-level feature perception image quality metrics, such as image local energy and phase consistency. Subsequently, the NSST inverse transform is applied to reconstruct the fused high-frequency and low-frequency components. This approach retains more image Enhanced Image Fusion Using Shearlet Transform and Pulse-Coupled Neural Networksthe proposed fusion framework outperforms existing methods in infrared-visible image fusion.