Advancements and Challenges in Visual SLAM: Process, Types, and Future Directions
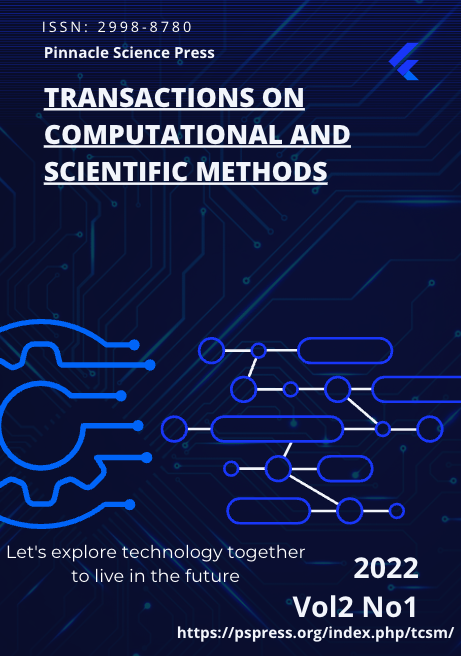
Abstract
Simultaneous Localization and Mapping (SLAM) has been a prominent research area in computer vision and mobile robotics for over two decades. Visual SLAM, which utilizes a camera as the sole external sensor, aims to create a map of an unknown environment while simultaneously determining the position of the sensors within that map. This paper provides an overview and synthesis of the visual SLAM process, key research findings in the field, and the available public datasets for visual SLAM. The paper also explores future directions in visual SLAM, including SLAM in dynamic environments, SLAM with multi-feature fusion, SLAM with multi-sensor integration, SLAM with multi-robot collaboration, and SLAM incorporating deep learning techniques.